Organic Perspectives of Knowledge Management:
Knowledge Evolution through a Cycle of
Knowledge Liquidization and Crystallization1
Koichi Hori
(Research Center for Advanced Science and Technology, University of Tokyo,
Japan
hori@ai.rcast.u-tokyo.ac.jp)
Kumiyo Nakakoji
(Research Center for Advanced Science and Technology, University of Tokyo,
Japan
kumiyo@kid.rcast.u-tokyo.ac.jp)
Yasuhiro Yamamoto
(Research Center for Advanced Science and Technology, University of Tokyo,
Japan
yxy@computer.org)
Jonathan Ostwald
(University Corporation for Atmospheric Research, Boulder, CO, USA
ostwald@ucar.edu)
Abstract: Our research on knowledge management is rooted in the
community perspective. We believe that knowledge systems should serve primarily
to help people create and share new knowledge. But we also acknowledge
the role of stable, structured and reliable information, both as a component
of our systems and as a component of the organizations within which we
work. The contribution of the paper is a framework for integrating organizational
and community perspectives on knowledge management and its computational
support. Our basic idea is that knowledge is not a static chunk of information,
but rather, knowledge evolves in a cycle of knowledge liquidization and
crystallization. The evolving process takes place through the interactions
among conceptual worlds, representational worlds, and the real world. This
paper first describes the knowledge liquidization and crystallization framework.
We then illustrate the approach with three systems, Knowledge Nebula Crystallizer,
livingOM, and ART-SHTA.
Keywords: knowledge liquidization and crystallization, knowledge
management, community of practice, Knowledge Nebula Crystallizer, livingOM,
ART-SHTA, organic perspective
Categories: K.6, H.3, H.5.3, H.5.4, I.2.4, I.2.6
1A
short version of this article was presented at I-Know '03, (Graz, Austria,
July 2-4, 2003).
1 Introduction
This paper presents our approach for the design and development of computational
support for knowledge work in organizations. Our conceptual framework has
evolved around organic perspectives of knowledge management.
We view knowledge not as a static chunk of information. But rather,
knowledge evolves in a cycle of knowledge liquidization and crystallization.
Knowledge does not exist as a static entity, but emerges only within a
certain context through interactions among conceptual worlds, representational
worlds, and the real world [Hori 1994]. Knowledge
can only be partially represented as a snapshot of such interactions. Represented
knowledge then serves as a seed for the production of new knowledge [Ostwald
1996][Nakakoji et al. 1998].
We have developed the knowledge liquidization and crystallization framework
based on the above viewpoint. The next section describes the framework.
2 The Knowledge Liquidization and Crystallization
Framework
The knowledge liquidization and crystallization framework integrates
organizational and community perspectives on knowledge management and its
computational support.
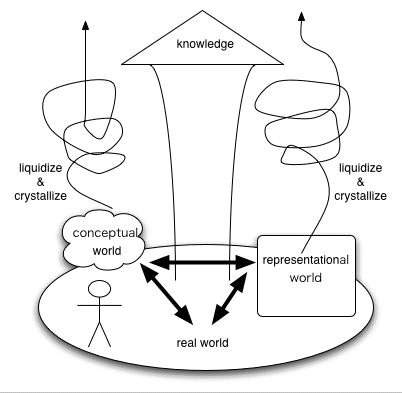
Figure 1: Knowledge evolves in the field of interaction among
conceptual world, representational world and real world.
Knowledge evolves in a cycle of knowledge liquidization and crystallization.
The evolving process takes place through the interactions among conceptual
worlds, representational worlds, and the real world (Figure
1). In the real world, real objects (including humans) exist and certain
relations hold among them. In a representational world, there exist words
and texts in natural languages and other forms of representations including
diagrams, graphs and pictures. A conceptual world exists in the human mind
and concepts are formulated there.
Let us now describe the process by using what we have found in analyzing
the protocol data of conversations between sales clerks and customers in
clothing stores [Shoji, Hori 2003].
In a clothing store, a good sales clerk often develops stories for his/her
customer about the customer's wearing the clothes to be purchased; for
instance, how the customer would look and be recognized in a wedding party.
Thus, a good sales clerk does not only sell a clothing product to the customer
but also tell the value or the meaning associated with the product by using
stories.
Such stories emerge while the sales clerk communicating with the customer.
The sales clerk constructs a story not by merely using his/her knowledge
about the product, but also by taking into account the customer's context.
Once developed, such a story becomes a part of the knowledge about the
product. By listening to the story, the customer's context also changes.
A story of attending a wedding party wearing the jacket to be purchased
was constructed in the representational world as a story. The story evoked
other concepts in the conceptual world, which were represented in some
utterances. They then evoked the search for the corresponding objects or
relations in the real world. The knowledge around the jacket (i.e., the
value or the meaning of the jacket) emerged in this evolution of the relations
among the conceptual world, the representational world, and the real world.
In order for knowledge to evolve in this manner, we argue that both
conceptual worlds and representational worlds need to go through an iterative
cycle of liquidization and crystallization.
Traditional approaches in knowledge management are to accumulated as
many coherent knowledge units as possible, and generalize them into a cohesive
structure. Then, the generalized knowledge can be instantiated when encountering
new situations. We argue that these approaches cannot afford dealing with
emerging contexts. Knowledge units can be accumulated in a coherent manner
only within a certain context. Generalized knowledge therefore is operational
only within this context.
Our knowledge liquidization and crystallization framework addresses
this issue. The idea is very simple. Knowledge liquidization decomposes
a knowledge representation into atomic units. Knowledge crystallization
identifies new relationships among some of the units capturing an emerging
context. That is, in contrast with knowledge generalization and instantiation,
knowledge liquidization divides a cohesive structure into coherent units
while knowledge crystallization discovers a new cohesive structure among
coherent units. In order to drive this discovery process, the framework
demands the involvement of human interaction in the process [Hori
94][Nakakoji et al. 98].
The next section presents our approach of the use of computational support
for the framework.
3 Systems for Knowledge Liquidization and Crystallization
Our approach is to design and develop computer systems that serve as
fields for the knowledge evolution based on the framework described above.
Such a computer system provides the user with the world of representation,
where the system makes certain calculation on the relations among the representations
and the user looks at and operates on the representations. The user can
develop his/her own conceptual world by finding new relations in the real
world or in the conceptual world through the operation of the representations.
The result of the concept formation is reflected again as new representation.
3.1 Requirements for the Systems
There are three requirements for the systems that are based on the knowledge
liquidization and crystallization framework.
- The representation should be divided into small elements to enable
new structuring.
- Each representation element should have rich possible connections with
other representation elements.
- The structured representation should leave enough room for the user
to imagine new concepts.
If the knowledge is represented in a fixed large chunk, it will be difficult
to consider other possibilities of new knowledge. Therefore, our system
first divides the knowledge into small elements in terms of a certain context.
This process is knowledge liquidization. Knowledge liquidization
can be achieved by both (1) decomposing a knowledge representation into
atomic units, and (2) adding links among atomic units of a knowledge representation.
A liquidized knowledge representation has a more flexible, "softer,"
malleable structure.
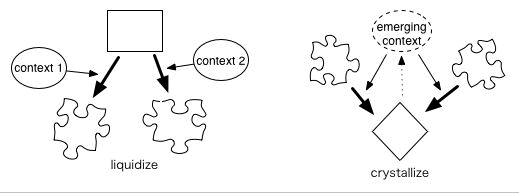
Figure 2: Components and Operations of the KNC
When liquidized, each element should have rich possible connections
with other elements. These two properties of fine granularity and rich
possible connections become the source of the evolution of new knowledge.
The system can calculate possible new structures using the possible connections.
This process is knowledge crystallization.
The result of the crystallization should not necessarily be some correct
answers but should evoke new concepts in the user's mind effectively. The
result of the crystallization should leave enough room for the user to
imagine new concepts. These operations are depicted in Figure
2.
A context in Figure 2 serves as a solvent for liquidization
(helping to divide artifacts into elements) and an emerging context serves
as a catalyst for crystallization (helping to retrieve and structure elements
into artifacts). A context determines the relation between elements and
artifacts, and can be viewed as domain knowledge.
We have been building computational prototype systems based on the above
framework to demonstrate several fundamental knowledge management processes
including knowledge reuse, creation, and evolution. In what follows, we
present some of our systems to illustrate the framework.
We first describe Knowledge Nebular Crystallizer, which is depicted
with a user scenario. We then describe livingOM and ART-SHTA to further
illustrate specific aspects of the approach.
3.2 Knowledge Nebula Crystallizer
The first prototype is called the Knowledge Nebula Crystallizer (KNC).
The KNC is a demonstration of concept developed at the University of Tokyo.
It is used in this paper to describe basic knowledge system functionalities
and components. In the scenario below we describe how an idealized version
of the prototype might work.
Jane is a graduate student who is developing her initial ideas for her
master's thesis. Her first step is to look, from her own viewpoint, into
the work her research group has done in the past. She logs into the Knowledge
Nebula Crystallizer (KNC), and tells the system of her interests. She also
uploads a 2-page description of her thesis topic to further describe what
information she is after.
The screen goes blank except for a small logo signifying that the KNC
is working to collect and crystallize (compile in various possible contexts)
information relevant to Jane's research interests. After about 10 seconds
the screen is filled with several different editions of reports summarizing
the relevant work selected from among the entire body of work done by the
research group; each edition shows her a different possible context or
a viewpoint discussing the same topic. The reports are organized from the
divided parts of the papers her group has produced that are relevant to
her interests. The KNC also provided a list of outside references that
were most often cited by her group as well as a list of the most prominent
researchers in her field, according to the possible different contexts.
The KNC has not written Jane's thesis, but it has certainly given her
a good start on the related work section, and stimulated her to consider
possible different contexts to discuss the same topic. It not only saved
her substantial time in collecting the material she needs for her own research,
but also triggered creating new knowledge.
We now jump ahead 6 months. Jane has finished her thesis. She once again
logs into the KNC only this time she is contributing knowledge rather than
consuming it. The KNC provides a simple form into which Jane uploads her
thesis as a TeX document. The screen KNC again goes blank except for the
liquidizing logo. After about 30 seconds a message appears on the screen
thanking Jane for her contribution. The KNC has successfully liquidized
and integrated Jane's thesis into its knowledge base, and is now better
able to help future users who request information related to Jane's topic.
The KNC may now give the new user the parts of information Jane gave combined
with other information under possibly new contexts that are different from
the context of Jane's master thesis.
In the scenario, the knowledge nebula evolved as Jane input her thesis
into the system. In this sense, the KNC is able to grow and improve over
time as the result of the work of users.
The above scenario was a fictionalized account of how the Knowledge
Nebula Crystallizer might contribute to knowledge sharing and knowledge
construction in a particular organization or community. The scenario depicted
two interactions with the user. In the first it output reports based on
Jane's query and in the second it accepted Jane's finished thesis. These
interactions involve the two essential operations of the KNC, Crystallization
and Liquidization, and the repository of the KNC, called the Knowledge
Nebula.
3.3 LivingOM
The second prototype is the livingOM (living Organizational Memory),
a web-based knowledge system developed at the University of Colorado at
Boulder. The livingOM shares the basic functionalities of the KNC, but
differs in its implementation and application. The role of the livingOM
in this paper is to illustrate how aspects of both community of practice
as well as organization perspectives can live together within a knowledge
system.
The livingOM supports work within a community of knowledge workers who
create, collect, and reuse information resources. It is living in the sense
that it grows and improves as it is used to do work and solve problems.
The livingOM is a follow-on to the DynaSites system [Fischer,
Ostwald 2001; Fischer, Ostwald 2002a; Fischer,
Ostwald 2002b], and has been conceptualized and developed as an organizational
memory serving a mid-sized research group. It is designed to both support
work practices and accumulate the products of work, such as research papers,
glossaries of terms, and information about group members and research prototypes.
The livingOM is web-based and is currently serving as the web site for
the enTWIne research group at the University of Colorado [enTWIne
2004].
Like the KNC, the livingOM manipulates information at both the artifact
and the element levels of granularity. Artifacts in the livingOM are hypertext
documents, whose elements (such as chapters, sections and paragraphs) are
structured hierarchically. A typical way to create a document in the livingOM
is to import a document written in MS-Word. The Word document is automatically
liquidized into elements and stored in the livingOM. Unlike the KNC the
elements are not through of as a nebula, although cross-document searches
are supported which return lists of elements.
The livingOM's domain knowledge plays a similar role to the domain knowledge
in KNC. Namely, it allows the system to collect and structure information
in response to a specific situation, or query. The representation of domain
knowledge, however, is different. Where the KNC represented domain knowledge
by high-dimensional vectors, the livingOM represents domain knowledge as
typed objects connected by relations.
Relations can be either explicitly created by users or automatically
created by the system. For example, a livingOM document contains relations
to resources such as glossary terms, citations, prototypes, and people
that are displayed as hypertext links embedded in the text.
When users traverse one of these links they access detailed information
about the particular resource (such as the definition of a glossary term)
as well as links to other related resources throughout the livingOM information
space.
Table 1 compares the two approaches of KNC and livingOM in support of
the knowledge liquidization and crystallization.
|
KNC |
livingOM |
Artifacts
(crystallized Elements) |
Documents stored by users and ones dynamically created |
Document stored by users and views that link related parts of documents
and domain knowledge |
Elements
(liquidized Artifacts) |
Nebula - information elements created from documents and interview
transcripts (but they could be created in anyway) |
Word documents are automatically decomposed to the paragraph level
(but they could also be created piece by piece) |
Domain Knowledge |
Thesaurus - Hand crafted set of keywords with automatically calculated
weight values against different contexts. There is also knowledge of people |
Several types of information, including Glossary, references, people,
research prototypes |
Table 1: Comparing KNC and livingOM
3.4 ART-SHTA
As noted in Section 2, the framework assumes the
involvement of human interactions with the system. By interacting with
liquidized knowledge representations, humans are to take some parts in
identifying structures among elements in the crystallization process. The
system's interaction design plays a key role in the process.
This subsection gives a brief overview of the ART-SHTA (Sculptural HyperText
Authoring) system to illustrate requirements for the visual interaction
design for the systems based on the knowledge liquidization and crystallization
framework.
ART-SHTA (Figure 3) supports information- and concept-structuring
for analytical and scholarly compositional tasks. ART-SHTA employs three
hypertext techniques: sculptural hypertext [Bernstein
et al. 2001], spatial hypertext [Marshall, Shipman
1995], and hierarchy browsing. The system has been used for such tasks
as to understand and specify system requirements, and to clarify research
concepts to design a structure for authoring an academic paper.
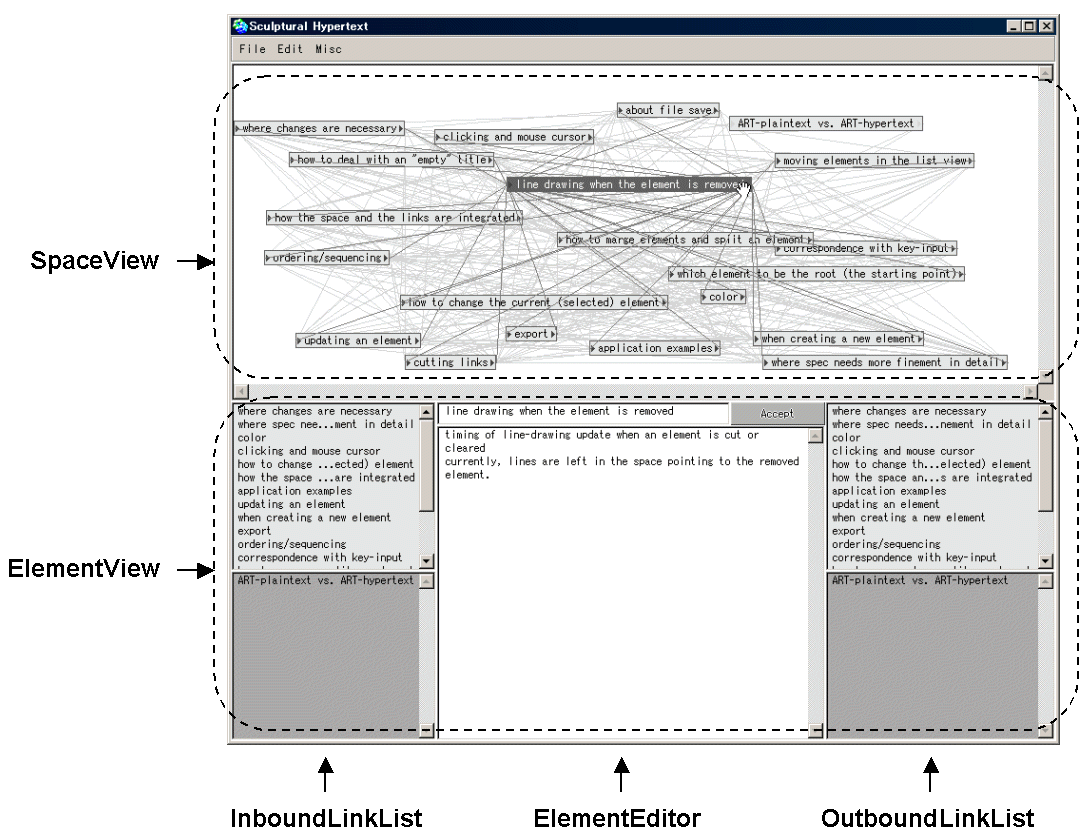
Figure 3: ART-SHTA
Sculptural hypertext refers to a type of hypertext where one "creates
a structure by removing unwanted connections, much as a sculptor may create
objects by removing unwanted material" [Bernstein
et al. 2001]. While the notion of sculptural hypertext was originally
explained to provide "exotic tools for hypertext narratives"
[Bernstein et al. 2001], we argue that the approach
also demonstrates a powerful method for supporting analytical and scholarly
authoring tasks, which we call sculptural hypertext authoring.
Spatial hypertext representations help users gradually define and fix
relationships among objects using emerging structures [Marshall,
Shipman 1995]. Additionally, with the interaction design technique
used in the four ART systems we have developed [Yamamoto
et al. 2002], a space provides a way to view the entirety, giving a
global view of the space of information chunks being constructed.
The 3-column view for hierarchy browsing has been used to display a
focused element in the central column, and linked elements in the columns
on the both sides. This allows a user to easily move within the hierarchy
without losing the context.
The ART-SHTA system has a name starting with "ART" because
throughout the interaction design of this system and other systems (such
as ART#001, #002, #003 and #004 presented in [Yamamoto
et al. 2002]), the design principle called ART (Amplifying Representational
Talkback) has been applied. ART emphasizes that computational media should
(1) allow a user to easily represent what he/she wants to externalize,
(2) allow a user to easily understand what he/she has externalized, and
(3) "be quiet," not offering disturbing services.
4 Discussion
To consider the entire lifecycle of knowledge management systems, Fischer
proposes the seeding, evolutionary growth, reseeding (SER) Model [Fischer
1998]. Our knowledge liquidization and crystallization framework can
be viewed as a framework for the evolutaionry-growth stage of the SER model.
The SER model provides a conceptual framework to understand how decentralized
evolution can be initiated and then sustained over time. The model was
developed to understand the balance between centralized and decentralized
evolution in sustained development of large systems. Its goal is to apply
lessons learned from success cases, such as the open source software, to
domains and communities, such as Knowledge Management, that have not traditionally
been viewed in from this perspective [Fischer, Ostwald
2001].
The knowledge our systems use to crystallize elements into artifacts
is implicit in the artifacts, rather than represented explicitly. There
is no notion of complete or correct in our systems, only more or less relevant
according to the crystallization method. The reliability of the artifacts
is assumed to be high since it is under control of the community, but in
any case the validity of the information is determined by the user, not
the system. These attributes mark our approach as belonging to the Community
of Practice perspective of knowledge management.
As Table 2 illustrates, organizations and community of practice seem
to have different perspectives on knowledge.
Organization |
Community of Practice |
Static (Centralized) |
Dynamic (Decentralized) |
Information-oriented |
Process-oriented |
Explicit (about the work) |
Implicit (the work itself) |
Table 2: What is knowledge? Two perspectives
By looking at the perspective of knowledge liquidization and
crystallization, however, we have come to a conclusion that
organizations and communities are not always so far apart. Modern
organizations need to see themselves not only as keepers of knowledge,
but also as creators of knowledge. And communities of practice,
likewise, have a real interest in sustaining their practice and
providing centralized resources to their users. We believe both
organizations and communities would benefit from an understanding of
their counterpart's perspective, and hopefully this paper has provided
a framework for doing so.
References
[Bernstein et al. 2001] Bernstein, M. (2001) Card
Shark and Thespis: Exotic Tools for Hypertext Narrative, Proceedings of
Hypertext 2001, ACM Press, Arhus, Denmark, pp.41-50.
[enTWIne 2004]enTWIne (2004) The enTWIne Project
Home Page, Available at http://www.cs.colorado.edu/~l3d/entwine.
[Fischer 1998] Fischer, G. (1998) Seeding, Evolutionary
Growth and Reseeding: Constructing, Capturing and Evolving Knowledge in
Domain-Oriented Design Environments, Automated Software Engineering, Vol.5,
No.4, pp. 447-464.
[Fischer, Ostwald 2001] Fischer, G., Ostwald,
J. (2001) Knowledge Management: Problems, Promises, Realities, and Challenges,
IEEE Intelligent Systems, January/February 2001, pp. 60-72.
[Fischer, Ostwald 2002a] Fischer, G., Ostwald,
J. (2002a) Seeding, Evolutionary Growth, and Reseeding: Enriching Participatory
Design with Informed Participation, Proceedings of the Participatory Design
Conference (PDC'02), Malme University, Sweden, pp. 135-143.
[Fischer, Ostwald 2002b] Fischer, G., Ostwald,
J. (2002b) Transcending the Information Given: Designing Learning Environments
for Informed Participation, Proceedings of ICCE 2002 International Conference
on Computers in Education, Auckland, New Zealand, pp. 378-381.
[Hori 1994] Hori, K. (1994) A System for Aiding
Creative Concept Formation, IEEE Transactions on Sytems, Man, and Cybernetics,
Vol.24, No.6, pp. 882-894.
[Marshall, Shipman 1995] Marshall, C.C., Shipman,
F.M. (1995) Spatial Hypertext: Designing for Change. in Communications
of the ACM, pp.88-97.
[Nakakoji et al. 1998] Nakakoji, K., Yamamoto,
Y., Suzuki, T., Takada, S., Gross, M.D. (1998) Beyond Critiquing: Using
Representational Talkback to Elicit Design Intention, Knowledge-Based Systems
Journal, Elsevier Science, Amsterdam, Vol.11, No.7-8, pp.457-468.
[Ostwald 1996] Ostwald, J. (1996) Knowledge Construction
in Software Development: The Evolving Artifact Approach, Ph.D. Dissertation,
University of Colorado at Boulder.
[Shoji, Hori 03] Shoji, H., Hori, K. (2003) Creative
communication for chance discovery in shopping. New Generation Computing
Vol.21, pp.73-86.
[Yamamoto et al 2002] Yamamoto, Y., Nakakoji, K.,
Aoki, A. (2002) Spatial Hypertext for Linear-Information Authoring: Interaction
Design and System Development Based on the ART Design Principle, Proceedings
of Hypertext2002, ACM Press, pp.35-44, June.
|