An OWL Ontology of Set of Experience Knowledge Structure
Cesar Sanin
(University of Newcastle, Australia
Cesar.Maldonadosanin@Newcastle.edu.au)
Edward Szczerbicki
(University of Newcastle, Australia
Edward.Szczerbicki@Newcastle.edu.au)
Carlos Toro
(VICOMTech Research Centre, Spain
ctoro@vicomtech.es)
Abstract: Collecting, distributing and sharing knowledge in a
knowledge-explicit way is a significant task for any company. However,
collecting decisional knowledge in the form of formal decision events as
the fingerprints of a company is an utmost advance. Such decisional fingerprint
is called decisional DNA. Set of experience knowledge structure can assist
on accomplishing this purpose. In addition, Ontology-based technology applied
to set of experience knowledge structure would facilitate distributing
and sharing companies' decisional DNA. Such possibility would assist in
the development of an e-decisional community, which will support
decision-makers on their overwhelming job. The purpose of this paper is
to explain the development of .an OWL decisional Ontology built upon set
of experience, which would make decisional DNA, that is, explicit knowledge
of formal decision events, a useful element in multiple systems and technologies,
as well as in the construction of the e-decisional community.
Keywords: Knowledge Acquisition, Knowledge Representation Formalism
and Method, Artificial Intelligence, Information Systems Applications,
Semantic Networks
Categories: H.4,
I.2,
I.2.4,
I.2.6
1 Introduction
Since the word knowledge took place into the business argot,
managers have tried to make it part of their assets. Knowledge seems
to be an invaluable asset of incalculable significance and it has been
considered as the only true source of competitive advantage of a
company [Drucker, 95]. Thus, the focus of
managers has turned to knowledge administration and many companies
have invested huge amounts of money to research technologies that
facilitate control of all forms of knowledge. In consequence, the
means and the ability of acquisition of knowledge, through efficient
transformation of information, can make the difference between the
success and failure of a company in the competitive environment of
global economy [Sanin, 04] and knowledge
society.
However, knowledge society arrived and brought with it all the difficulties
that information faces. Characteristics such as unstructured, disintegrated,
not shareable, incomplete, and uncertain information represent an enormous
problem for information technologies (IT) [Ferruci, 04;
Deveau, 02]. Under these circumstances, the process
of transforming information into knowledge is critical and difficult, because
unfortunately, knowledge depends upon information [Sanin,
04]. Moreover, Awad and Ghaziri [Awad, 04] gives
acknowledge of another difficulty when affirms that up to 95 percent of
information is preserved as tacit knowledge (for tacit and explicit knowledge
see [Nonaka, 95]). Thus, one of the most complicated
issues about knowledge is its representation, because it determines how
knowledge is acquired and how knowledge is transformed from tacit knowledge
to explicit knowledge. Knowledge must be obtained and represented in an
understandable form for the agents that experienced it. Hence, it is evident
that some kind of technology is necessary to transform information into,
not just knowledge, but explicit knowledge.
One theory proposes that experienced decision-makers base most of
their decisions on situation assessments [Noble,
98]. In other words, decision-makers principally use experience
for their decisions, i.e. when a decision event emerges, managers
select actions that have worked well in previous similar
situations. Similar situations at thi stage operate at an abstract
level. Nevertheless, when applying similarity in a realistic level, it
depends upon the knowledge representation used. Then, managers, in a
not yet well known brain process, extract the most significant
characteristics from the current circumstances, and relate them to
similar situations and actions that have worked well in the
past. However, what it is clear here is that it is very important to
keep a record of earlier decisions events. In consequence, tools for
representing and storing formal decision events in a
knowledge-explicit way are evidently necessary, understanding that a
formal decision event is a decision occurrence that was made following
procedures that make it structured and formal [Sanin, 05a].
Many technologies, such as Knowledge Management Systems (KMS), Data
Mining (DM) and Knowledge-base Systems (KbS) among others, are
currently working with different types of knowledge. Moreover,
although these technologies work with decision-making in some
approaches, they do not keep structured knowledge of the formal
decision events they participate on. For us, any technology able to
capture and store formal decision events as explicit knowledge will
improve the decision-making process, reducing decision time, as well
as avoiding repetition and duplication in the process. Unfortunately,
computers are not as clever as to form internal representations of the
world, and even simpler, representations of just formal decision
events. Instead of gathering knowledge for themselves, computers must
rely on people to place knowledge directly into their memories. This
problem suggests deciding on ways to represent information and
knowledge inside computers. Set of Experience Knowledge Structure is
a combination of filtered information obtained from formal decision
events performed by different technologies, i.e. it acquires knowledge
from technologies that take structured decisions. Nevertheless,
despite the fact that set of experience knowledge structure is already
developed, the ways for acquiring its knowledge are supported on the
confidence of every technology that performs formal decisions [Sanin, 06b].
Set of experience knowledge structure has been developed as part of
a platform for transforming information into knowledge named Knowledge
Supply Chain System (KSCS) [Sanin, 05a]. KSCS performs
by manipulating sets of experience to keep formal decision events and to
help managers in the daily operation of decision-making. In brief, the
KSCS takes information from different technologies that make formal decision
events, integrates them and transforms them into knowledge making use of
sets of experience. Fully developed, KSCS certainly would improve the quality
of decision-making, and could advance the notion of administering knowledge
in the current decision making environment.
Once a structure such as set of experience allows constructing the decisional
DNA of a company, it is necessary to increase the means for sharing this
experience among different agents. Distributing decisional DNA, not just
inside a company, but also among many companies, would help on the establishment
of a knowledge sharing community, which, if it is developed through internet,
it would be called the e-decisional community, that is, a decisional Community
of Practice (CoP).
Different technologies can help in accomplishing such task, but no
one like Ontology-based technology, which offers differentiable
advantages. Ontology-based applications are probably the fields in
which computer-based semantic tools and systems are more extended
nowadays for several heterogeneous domains, mainly focused in querying
and classification purposes in information sharing and knowledge
management contexts. Ontologies are commonly used in artificial
intelligence and knowledge representation. Computer programs can use
Ontologies for a variety of purposes including inductive reasoning,
classification, a variety of problem solving techniques, as well as to
facilitate communication and sharing of information among different
systems. In addition, emerging semantic web systems use Ontologies
for a better interaction and understanding between different web-based
systems using agents. In this last direction, a recent survey of
Ontology-based applications, with focus on e-commerce, knowledge
management, multimedia, information sharing and educational
applications, can be found in Ramos and Gomez-Perez [Ramos, 04].
In conclusion, the purpose of this paper is to explore Ontologies
under the view of set of experience knowledge structure, leading onto
the creation of a new community of practice named e-decisional
community, which would share companies' decisional DNA. In such
way, set of experience Ontology-based knowledge structure would have
the potential to improve the way knowledge is managed as an asset in
current decision-making environments.
2 Background
Nature has developed potent tools to store and manage information and
knowledge. Firstly, DNA has been judged by many researchers as the most
excellent data structure. The survival of information in nature over successive
generations has showed the success of the DNA structure, and its mechanisms.
DNA stores information for survival of the species, and allows for improvement
through evolution. Secondly, the brain has been considered as the most
powerful processor and database. The brain stores knowledge in terms of
keeping experience from past situations, as well as knowledge from preventive
experience of others, i.a. a cultural knowledge. This stored knowledge
is used for the survival of the individual, and the improvement of its
existence [Sanin, 05a].
Two potent natural structures created for the survival of the group
and the individual showing that one of the most valuable intellectual assets
are the experiences accumulated during processes.
2.1 Deoxyribonucleic Acid (DNA)
DNA is a nucleic acid found in cells that carries genetic information,
and is the molecular basis of heredity. DNA is made from two strands that
stick together with a slight twist; it looks like two strands forming a
helical spiral winding around a helix axis. Each strand is a long combination
of four basic elements called nucleotides. These nucleotides are Adenine
(A), Thymine (T), Guanine (G) and Cytosine (C). Their combination allows
for the different characteristics of each individual, and becomes as one
of the highlighted uniqueness of this kind of structure. One part of the
long strand comprises a gene. A gene is a portion of a DNA molecule, which
guides the operation of one particular component of an organism. Genes
give orders to a living organism about how to respond to different stimuli.
Finally, a set of genes makes a chromosome, and multiple chromosomes make
the whole genetic code of an individual [see Fig. 1].
For a deeper information please refer to [Miller, 02].
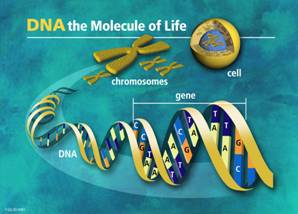
Figure 1: DNA the Molecule of Life (Image credit U.S.
Department of Energy Human Genome Program (http://www.ornl.gov/hgmis)
DNA demonstrates unique aspects as a data structure [Ryu,
04]. Information about the living organism is kept to be passed on
to future generations, as well as being the basis of new elements in the
organism which are evaluated in terms of performance. DNA stores information
for the survival of the species, and improvement in the evolutionary chain.
2.2 The Brain
The brain, as the organ of the central nervous system of vertebrates,
controls thought and neural coordination. It receives stimuli from the
sense organs, and combines and interprets them to formulate a response.
Psychologists have tried to explain how the brain acts and keeps experience,
and most important, how it takes decisions. One of these psychologists,
George Kelly, established a theory and introduced the idea of a psychological
space as a term for an imaginary region in which we may place and classify
elements of our experience. This space does not pre-exist, and in consequence,
each individual goes through a process of construction. This space will
provide a kind of topological system of personal experience [Shaw,
92]. Hence, the theory proposes that experience is kept in our brain
in the way of multiple correlated variables describing a situation. Decisions
events are part of these situations that can be kept in the psychological
space. Experience is, possibly, stored in the brain in the way Kelly proposes,
and once the psychological space is constructed, it can be used in the
way that Noble [Noble, 98] proposes decision-makers
operate.
Experienced decision events of the living organism are kept in the brain
for future situations. The brain stores experience for the survival of
the individual, and improvement of its own existence.
2.3 Current Trends in Knowledge and Data Structures
From a mechanistic point of view, reasoning in machines is a computational
process. This computational process, to be feasible, definitely needs systemic
techniques and data structures, and in consequence, several techniques
have been developed trying to represent and acquire knowledge. Although,
every representation must be implemented in the machine by some data structure,
the representational property is in correspondence to something in the
world, says a decision event, and in the constraints imposed by
association [Sanin, 05a].
A Knowledge Representation (KR) is fundamentally a replacement, a
substitute for the thing itself. It is used to allow determining
consequences by thinking rather than acting, i.e. by reasoning about
the world rather than taking action in it. Moreover, a KR is a medium
of human expression, i.e. a language in which we say things about the
world. It is an element of intelligent reasoning, a medium for
organizing information to facilitate making inferences and
recommendations, and a set of ontological commitments, i.e. an answer
of how to interpret the world [Davis, 93].
KR looks to be equally useful for intangible objects as well as
tangible objects. Davis, Shrobe, and Szolovits expressed that
"representations function as surrogates for abstract notions such
as actions, processes, beliefs, causality, and categories" [Davis, 93], and therefore, representations can be
also used on formal decision events.
In past years, many knowledge representation structures have been
developed. Logic, rules, and frames appear as the most generalized
techniques, and symbolize the kinds of things that are important in
the world; even though developed systems can used exclusively one of
the techniques, their hybridization is a common element. Logic
implicates understanding the world in terms of individual entities and
associations between them.
Rule-based systems view the world in terms of attribute-object-value
and the rules that connect them. Frames, on the other hand, comprise
thinking about the world in terms of prototypical concepts. Hence,
each of these representation techniques supplies its own view of what
is important to focus on, and suggests that anything out of this focus
may be ignored [Davis, 93].
Recent advances in the field of KR have converged on constructing a
Semantic Web, an extension of the current World Wide Web, looking for
publishing information in a form that is easily inferable to both
humans and machines. Current progresses have led to the
standardisation of the Web Ontology Language (OWL) by the World Wide
Web Consortium (W3C). OWL provides the means for specifying and
defining ontologies — collections of descriptions of concepts in
a domain (classes), properties of classes, and limitations on
properties. OWL can be seen as an extension from the frame based
approach to knowledge representation, and a division of logic called
Description Logics (DL) [Schatz, 04].
All these possible representations can be used for many purposes,
and those points of view are useful, but they are not the only
ones. Only finite representations of something are available, never
something complete. Sokolowski observes, "the thing can always be
presented in more ways than we already know; the thing will always
hold more appearances in reserve" [Tsoukas,
04, p.4].
3 Set of Experience Knowledge Structure
Knowledge is a fluid mix of experiences expressed in terms of
values, related information, and expert insight, which provides a
framework for evaluating and incorporating new experiences and
information [Coakes, 03].
As was said above, one of the most valuable intellectual assets is the
experience accumulated during processes, and in our case, the experience
acquired in making a decision. Based upon Kelly's theory of psychological
space, we develop a knowledge structure to manage formal decision
events, a structure that builds up this space with formal decision
experiences.
Set of experience has been developed to store formal decision
events in an explicit way. It is a model based upon existing and
available knowledge, which must adjust to the decision event is built
from. Four basic components surround decision-making events:
variables, functions, constraints, and
rules. They are stored in a combined dynamic structure that
comprises set of experience [see Fig. 2]. In this
text a concise idea of set of experience and its components is
offered, for additional information Sanin and Szczerbicki [Sanin, 05a] should be examined.
Variables usually involve representing knowledge using an
attribute-value language (that is, by a vector of variables and
values) [Lloyd, 03]. This is a traditional
approach from the origin of knowledge representation, and is the
starting point for set of experience. Variables that intervene in the
process of decision-making are the first component of the set of
experience. These variables are the centre root of the structure,
because they are the origin of the other components.
Based on the idea of Malhotra [Malhotra, 00]
who maintains that "to grasp the meaning of a thing, an event, or
a situation is to see it in its relations to other things",
variables are related among them in the shape of functions. Functions,
the second component, describe associations between a dependent
variable and a set of input variables; moreover, functions can be
applied for reasoning optimal states, because they come out from the
goals of the decision event. Therefore, set of experience uses
functions, and establishes links among the variables constructing
multiobjective goals.
According to Theory of Constraints (TOC), Goldratt [Goldratt, 86] affirms that any system has at least
one constraint; otherwise, its performance would be infinite. Thus,
constraints are another way of relationships among the variables; in
fact, they are functions as well. A constraint, as the third
component of set of experience, is a restriction of the feasible
solutions in a decision problem, and a factor that limits the
performance of a system with respect to its goals.
Finally, rules are suitable for associating actions with conditions
under which the actions should be performed. Rules, the fourth component
of set of experience, are another form of expressing relationships among
variables. They are conditional relationships that operate in the universe
of variables. Rules are relationships between a condition and a consequence
connected by the statements IF-THEN-ELSE.
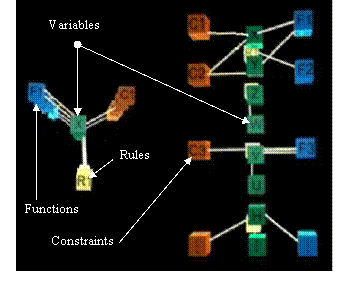
Figure 2: Structure of the Set of Experience
In conclusion, the set of experience consists of variables,
functions, constraint and rules, which are uniquely combined to
represent a formal decision event. Set of experience can be used in
platforms to support decision-making, and new decisions can be made
based on sets of experience.
Following the description of the four components of set of
experience, its structure is organized taking into account some
important features of DNA. Firstly, the combination of the four
nucleotides gives uniqueness to DNA, just as the combination of the
four components of the set of experience offer
distinctiveness. Moreover, the elements of the structure are connected
among themselves imitating part of a long strand of DNA, that is, a
gene. Then, a gene can be assimilated to a set of experience, and, in
the same way as a gene produces a phenotype, a set of experience
produces a value of decision in terms of its objective functions. This
value of decision is what is called the efficiency of the set
of experience. The efficiency or phenotype value is a combination of
the objective functions and the effect values of the variables [Sanin, 05a].
Furthermore, it is possible to group sets of experience by
category, that is, by their phenotype or efficiency. Each set of
experience built after a formal decision event can be categorized, and
acts as a gene in DNA. A gene guides hereditary responses in living
organisms. As an analogy, a set of experience guides the responses of
certain areas of the company about future decisions. For instance, a
formal decision took effect in the production area about a production
quantity; the set of experience formed after this decision becomes a
gene for the area and could help to shape future decisions in the same
field. The sets of experience give advice to the company's areas about
how to respond.
Additionally, suppose two formal decision events have the same
characteristics in terms of structure and category, i.e. same
variables, functions, constraints, and rules [Sanin,
06c], but, are slightly changed in the efficiency value, then both
sets of experience can originate a new improved and more precise set
of experience. They are transformed achieving an improvement due to a
mixing of the efficiency of the sets of experience. It acts as if two
similar parent genes are mixed up to obtain a unique improved
gene. This possibility opens doors for reformulating sets of
experience.
According to Kelly [Shaw, 92], a unique
personal construct (a set of experience) is appropriate only for the
anticipation of a finite number of events, and the psychological space
is never a complete system, then one set of experience cannot rule a
whole system, even in a specific area or category. Therefore, more
sets of experience should be acquired and constructed. The day-to-day
operation provides many decisions, and the result of this is a
collection of many different sets of experience. Hence, a group of
sets of experience of the same category comprise a kind of chromosome,
as DNA does with genes. These chromosomes or groups of sets of
experience could make a "strategy" for a category, i.e. an
area of the company. They are a group of ways to operate when making
decisions. Each module of chromosomes forms an entire inference tool,
and provides a schematic view for knowledge. Such complete group is
what we called the Decisional DNA of the company.
In conclusion, set of experience knowledge structure acts as a
representation for explicit knowledge according to the world it
perceives from formal decision events. It is composed by four
components, which are uniquely combined. Sets of experience can be
collected, classified, and organized according to their efficiency,
grouping them into chromosomes. Chromosomes are groups of sets of
experience that can comprise a strategy for a specific area of the
company. Moreover, improvements can be made upon some sets of
experience by transformations and groups of chromosomes are the
decisional DNA of the company.
4 Ontology-based Technology
Let us recall shortly what Ontologies are and how they are used. In
philosophy, Ontology is the most fundamental branch of metaphysics. It
studies being or existence, as well as the basic categories thereof,
that is, tries to find out what entities and what types of entities
exist. However, in the Computer Science domain there is a different
definition.
The following is a Tom Gruber's widespread accepted definition of
what Ontology is in this context: Ontology is the explicit
specification of a conceptualization; a description of the concepts
and relationships in a domain [Gruber, 95].
Nowadays, probably the fields in which computer-based semantic tools
and systems are more extended are Ontology-based applications for several
heterogeneous domains, for instance: medical (LinkBase), chemical (ChEBI
BAO), legal (LODE), cultural (CIDOC-CRM), among others. They mainly focus
in information sharing and knowledge management contexts for querying and
classification purposes.
It is true, however, that many researchers in the AI society start their
publications with their own definitions of Ontologies, but in short, the
definition above is well accepted. Thus, in the context of AI, we can describe
the Ontology of a program by defining a set of representational terms.
In such Ontology, definitions associate names of entities in the universe
of discourse (e.g. classes, relations, functions, or other objects) with
human-readable text describing what the names mean, and formal axioms that
constrain the interpretation and well-formed use of these terms.
Ontologies are commonly used in artificial intelligence and
knowledge representation. Computer programs can use Ontologies for a
variety of purposes including inductive reasoning, classification, and
problem solving techniques, as well as communication and sharing of
information among different systems. In addition, emerging semantic
web systems use Ontologies for a better interaction and understanding
between different agent web-based systems. In this last direction, a
recent survey of Ontology-based applications, focused on e-commerce,
knowledge management, multimedia, information sharing and educational
applications, can be found in Ramos and Gomez-Perez [Ramos, 04].
Ontologies can be modelled using several languages, being the most
widely used RFD and recently OWL (both expressed in eXtensible Markup
Language-XML). OWL (Ontology Web Language), a W3C Recommendation
since February 2004 [W3C, 04], has been designed
to be used by applications that need to process content of information
instead of just presenting information to humans. OWL facilitates
machine interpretability of web content by providing additional
vocabulary along with formal semantics, and it is considered better
than XML, RDF, and RDF Schema (RDF-S). Moreover, OWL has three
increasingly-expressive sublanguages: OWL Lite, OWL DL, and OWL
Full.
One of the most widely reviewed problems arising when handling
large amount of data is to query the repository in an efficient
way. Such problem has been researched from the databases point of
view; however, one big handicap is the fact that every query must be
highly structured and well defined. Ontology modelling can deliver
interesting benefits as it allows inferring semantically new derived
queries. These queries relate concepts that were not taken into
account initially. Modern inference engines and reasoners like Pellet
and Racer [Sattler, 06] deliver a highly
specialized, yet efficient way to perform such queries via a JAVA
compliant API. In the literature, data handling by Ontology-based
technology is reported by researchers in fields such as Large Model
Visualization for industrial plants [Posada, 05],
Geographic information Systems [Rewerse, 05], and
the modelling of design stages and processes [Brandt,
06].
Furthermore, user modelling, task and experience are also possible scenarios
for the exploitation of semantic data by Ontology-based technology as it
was addressed for example in the IST-Project WIDE [Smithers,
04]. Moreover, by its own nature Ontologies provide a semantic point
of view over an XML approach as the query process of the shareable knowledge
structure is enhanced by the use of reasoners and semantic information
embedded in the system.
In conclusion, set of experience Ontology-based containing knowledge
about formal decision events can be a scenario for exploitation of semantic
data, and in such way, it can be used as a shareable structure for helping
in the decision-making process. Following, set of experience Ontology-based
knowledge structure is exposed.
5 Set of Experience Ontology-based Knowledge Structure
In this section, we introduce our approach to the modelling of sets
of experience knowledge structure from an Ontology perspective. In order
to obtain such Ontology, we start from the XML set of experience model
presented by Sanin and Szczerbicki [Sanin, 05b; Sanin,
06a], where they established an initial shareable model for set of
experience. Afterwards, an Ontology model process was performed using the
Protégé editor [Protégé, 05].
5.1 Class, Slots and Instances Organization
When developing Ontologies three actions must be taken into account:
- Initially a set of classes must be modelled with the elements of the
domain; these classes contain the abstract concepts and their roles as
concrete or abstract depending on the level of conceptualization desired.
- Every class has properties including name, cardinality (single or multiple)
and the data type that describes the property. In RDF, properties are called
slots; while in OWL, they are branded properties with the capability to
be data typed or object typed, that is, they can refer to other properties
and even classes or instances of the Ontology.
- When the Ontology modelling process is done, it must be instanced.
This process can be performed using an Ontology editor like
Protégé or programmatically via an API. The instancing
process populates every class and the relationships between them with
real world values. At this stage of our work we have modelled the
Ontology under an RDF approach.
5.2 Set of Experience Knowledge Structure modelling —
implementation — visualization
For every first level tag of the XML set of experience knowledge
structure a concrete class of the Ontology is created (role concrete),
that is, variables, functions, constraints, and rules, among
others. For second level tags, a slot with the proper cardinality and
data type is created. A tag from the XML version of set of experience
knowledge structure can be seen in [Fig. 3] (using
a free visualization tool for XML).
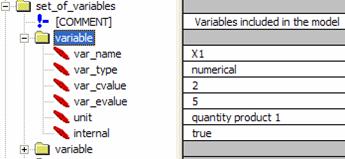
Figure 3: Tag Variable in the XML version of the Set of experience
Knowledge Structure
Same tag is shown in its Ontology perspective in [Fig.
4], while the Ontology instancing process using the Protégé
editor can be seen in [Fig. 5].
In [Fig. 3, 4 and 5],
the different stages of the variable class can be observed. Same process
was performed on the functions, constraints and rules classes.
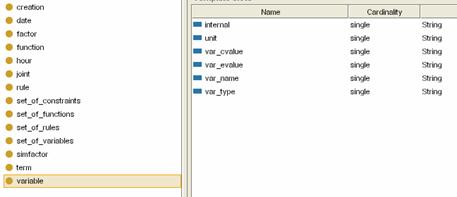
Figure 4: Tag Variable in the Ontology version of the Set
of experience Knowledge Structure
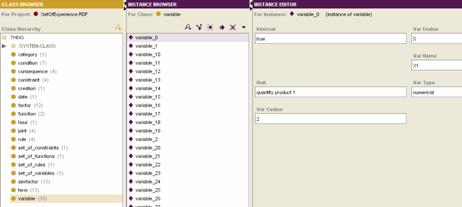
Figure 5: Tag Variable instanced in the Ontology version
of the Set of experience Knowledge
In [Fig. 6], relationships among the different classes
of the Ontology can be seen using a plug-in for visualization of the Ontology
model.
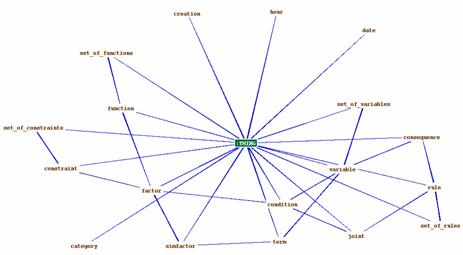
Figure 6: Ontology model relationships
Structural changes were not developed in the transformation of set
of experience XML-structure to set of experience OWL-structure. Having
finished first and second actions of the Ontology modelling process,
the third action continues as it is explain in the next section [Section 5.3].
5.3 Instancing Set of Experience Ontology-based
Knowledge Structure
Formal decision events can be evaluated via an Ontology API
(Protégé provides one which is based in JENA). Using
such API programmatically, the Ontology instantiation process can be
performed, that is, filling in the knowledge model with real world
values. Furthermore, the API provides several mechanisms to test
semantics on the conceptual model and the instanced model as
well.
The Ontology model by nature is a Web based application with a
predefined namespace. This permits storage of several instanced models
in a web server way, allowing the users to interact with the model
using a simple web browser, or in our case, a JAVA application for the
handling of the Ontology.
Once the Ontology is instanced, the model becomes a shareable explicit
knowledge that can be considered a repository.
Moreover and very important, the standardization of RDF and OWL by
the W3 consortium indirectly gives this process a sort of modelling
standardization, thus the instances themselves will be shareable among
users.
The model is now ready for instancing with companies' decisional
DNA, that is, formal decision events. A repository for sets of
experience according to the Knowledge Supply Chain System platform
exposed by Sanin and Szczerbicki in [Sanin, 04]
can be created. Once this is done, this repository can be accessed
through different queries, which would be developed according to
similarity parameters [Sanin, 06c] and users'
requirements.
Having an Ontology-based repository ready to be feed with
decisional DNA produced by the members of the e-decisional community
is the beginning of a new way of sharing knowledge. The e-decisional
community would share decisions among its members allowing
decision-maker users to improve their day-to-day operation by
consulting such repository, and along with this interaction, the
e-decisional community would increase and improve the decisional DNA
available for being shared.
Currently, a specific case of application is being implemented on
industrial design where set of experience Ontology-based knowledge
structure is used on the basis of creating decisional DNA for the
elements that comprise a plant and the users that control its
maintenance and revision. Besides, the KSCS is now approaching its
final developments which will put it through a process of
implementation by using set of experience Ontology-based knowledge
structure.
6 Conclusion
Improving set of experience knowledge structure from a XML-based
technology to an Ontology-based technology XML adheres heavier
semantics and logic, as well as more expresivness. A shareable
enhanced set of experience Ontology-based knowledge structure able to
store formal decision events, i.e. decisional DNA, would advance the
notion of administering knowledge in the current decision making
environment. Decisional DNA enables us to distribute experience among
different applications, and in that form, and through the e-decisional
community, companies that are expanding the knowledge management
concept externally, can explore new ways to put explicit classifiable
knowledge in the hands of employees, customers, suppliers, and
partners.
References
[Awad, 04] Awad, E. and Ghaziri, H.:
"Knowledge Management"; Pearson Education Inc., Prentice
Hall / New Jersey (2004).
[Brandt, 06] Brandt, S.C., Morbach, J.,
Miatidis M., et al: "Ontology-based information management in
design processes"; Proc. 16th European Symposium on Computer
Aided Process Engineering (ESCAPE) and 9th International Symposium on
Process Systems Engineering (PSE), Garmisch-Partenkirchen,
(2006).
[Coakes, 03] Coakes, E.: "Knowledge
Management: Current Issues and Challenges"; IRM Press / London
(2003).
[Davis, 93] Davis, R., Shrobe, H. and
Szolovitz, P.: "What is a Knowledge Representation?"; AI
Magazine, 14, 1 (1993), 17-33.
[Deveau, 02] Deveau, D.: "No brain, no
gain: Knowledge management"; Computing Canada, 28, (2002),
14-15.
[Drucker, 95] Drucker, P.: "The
Post-Capitalist Executive: Managing in a Time of Great Change";
Penguin / New York (1995).
[Ferruci, 04] Ferruci, D. and Lally, A.:
"Building an example application with the Unstructured
Information Management Architecture"; IBM Systems Journal, 43, 2
(2004), 455-475.
[Goldratt, 86] Goldratt, E.M. and Cox, J.: "The
Goal"; Grover / Aldershot, Hants (1986).
[Gruber, 95] Gruber, T.R.: "Toward
principles for the design of Ontologies used for knowledge
sharing"; International Journal of Human-Computer Studies, 43,
5-6 (1995), 907-928.
[Lloyd, 03] Lloyd, J.W.: "Logic for
Learning: Learning Comprehensible Theories from Structure Data";
Springer / Berlin (2003).
[Malhotra, 00] Malhotra, Y.: "From Information
Management to Knowledge Management: Beyond the 'Hi-Tech Hidebound' Systems";
Knowledge Management for the Information Professional, Information Today,
Inc., 37-61.
[Miller, 02] Miller, Kenneth, R. and Levin, J.:
"Biology"; Prentice Hall / Saddle River (2002).
[Noble, 98] Noble, D.: "Distributed Situation
Assessment"; Proc. FUSION '98 International Conference (1998).
[Nonaka, 95] Nonaka, I. and Takeuchi, H.: "The
Knowledge-Creating Company: How Japanese Companies Create The Dynamics
Of Innovation"; Oxford University Press / New York (1995).
[Posada, 05] Posada, J., Toro, C.A., Wundrak, S.,
Stork, A.: "Ontology Supported Semantic Simplification of Large Data
Sets of Industrial Plant CAD Models for Design Review Visualization";
Proc. Int. Conf. on Knowledge-base and Intelligent Information and Engineering
Systems, KES, Part I LNCS 3681, Springer, Melbourne (2005).
[Protégé, 05] Protégé.
Stanford Medical Informatics, http://protege.stanford.edu/index.html.
(2005).
[Ramos, 04] Ramos, J. and Gomez-Perez, A.: "A
survey on Ontology-based applications. e-commerce, knowledge management,
multimedia, information sharing and educational applications"; Public
deliverable of the EU-Project OntoWeb, January (2004).
[Rewerse, 05] Rewerse: "Ontology Driven
Visualisation of Maps with SVG — Deliverable from the European Project
REWERSE"; Project number: IST-2004-506779, Munich (2005).
[Ryu, 04] Ryu, W.: "DNA Computing: A Primer";
November, www.arstechnica.com/reviews/2q00/dna/dna-1.html.
(2004)
[Sanin, 04] Sanin, C. and Szczerbicki, E.: "Knowledge
Supply Chain System: A Conceptual Model"; Knowledge Management: Selected
Issues, A. Szuwarzynski (Ed), Gdansk University Press / Gdansk, (2004)
79-97.
[Sanin, 05a] Sanin, C. and Szczerbicki, E.: "Set
of Experience: A Knowledge Structure for Formal Decision Events";
Foundations of Control and Management Sciences, 3, (2005) 95-113.
[Sanin, 05b] Sanin, C. and Szczerbicki, E.: "Using
XML for Implementing Set of Experience Knowledge Structure"; Proc.
Int. Conf. on Knowledge-base and Intelligent Information and Engineering
Systems, KES, Part I LNCS 3681, Springer, (2005), 946-952.
[Sanin, 06a] Sanin, C. and Szczerbicki, E.: "Extending
Set of Experience Knowledge Structure into a Transportable Language XML
(eXtensible Markup Language)"; Cybernetics and Systems: An International
Journal, 37, 2-3 (2006), 97-117.
[Sanin, 06b] Sanin, C. and Szczerbicki, E.: "Using
Set of Experience in the Process of Transforming Information into Knowledge";
International Journal of Enterprise Information Systems, 2, (2006) 45-62.
[Sanin, 06c] Sanin, C. and Szczerbicki, E.: "Similarity
Metrics for Set of Experience Knowledge Structure"; Proc. Int. Conf.
on Knowledge-base and Intelligent Information and Engineering Systems,
KES, Part I LNAI 4251, Springer, (2006), 663-670.
[Sattler, 06] Sattler, U.: "Description Logic
Reasoners"; April, http://www.cs.man.ac.uk/~sattler/reasoners.html,
(2006).
[Schatz, 04] Schatz, B., Mohay, G. and Clark, A.:
"Rich Event Representation for Computer Forensics"; Proc. APIEMS
- Fifth Asia Pacific Industrial Engineering and Management Systems Conference,
QUT, Gold Coast (2004).
[Shaw, 92] Shaw, M. and Gaines, B.: "Kelly's
"Geometry of Psychological Space" and its Significance for Cognitive
Modelling"; The New Psychologist, October, (1992) 23-31.
[Smithers, 04] Smithers, T., Posada, J., Stork,
A., Pianciamore, M., et al.: "Information management and knowledge
sharing in WIDE"; Proc. European Workshop on the Integration of Knowledge,
Semantics and Digital, (2004).
[Tsoukas, 04] Tsoukas, H. and Mylonopoulos, N.:
"Organizations as Knowledge Systems: Knowledge, Learning and Dynamic
Capabilities"; Palgrave Macmillan / New York (2004).
[W3C, 04] W3C: "OWL Web Ontology Language Reference";
W3C Recommendation, 10 February 2004, http://www.w3.org/TR/2004/REC-owl-ref-20040210/.
(2004).
[Watson, 53] Watson, J. and Crick, F.: "A Structure
for Deoxyribose Nucleic Acid", Nature, April 171, (1953) 737-738.
|