The Impact of Behavioral Monitoring Technology on the
Provision of Health Care in the Home
Anthony P. Glascock
(Drexel University, USA
glascock@drexel.edu)
David M. Kutzik
(Drexel University, USA
kutzik@drexel.edu)
Abstract: The lack of appropriate and accurate information on
the ability of a frail individual to accomplish specific task oriented
activities can place the individual at risk or result in the allocation
of costly and unnecessary care. Although there have been previous attempts
to use computer technology to obtain this information, they have proved
to be costly and complex and therefore not widely used. However, a behavioral
monitoring technology, based on smart-home and telemedicine applications,
has been developed that obtains more accurate and timely information on
the ability of frail individuals to accomplish specific tasks in their
own residences than any other existing method. During a twelve month pilot
study, this system has been used by care providers to assess the status
of their clients, respond to immediate needs and alter overall care plans,
thus resulting in better care and greater peace of mind for the individual.
Keywords: Smart-home technology, Telemedicine, Behavioral monitoring,
Care provision, Web-based services
Categories: J.3
J.4, K.4.1, K.4.2
1 Introduction
The goal of all care for the frail elderly, whether provided formally
by professionals or informally by family members, is the delivery of the
appropriate intervention, by the appropriate person, at the appropriate
time. To achieve this goal, a clear and accurate knowledge of a person's
performance of functional tasks in their home environment is absolutely
necessary. The objective of health informatics, in particular pervasive
computing, is to find innovative means of applying technology in order
to extend health care beyond the conventional clinical setting to the individual
in their own residence within the community. Thus, there appears to be
a convergence of these two goals: to use the technological advances associated
with pervasive computing to assess changes in the functional abilities
of frail older people in their own homes in order to enhance their wellbeing
by providing needed services in a timely manner. We report on one such
attempt to use recent breakthroughs in smart-home technology and the Internet
to provide vital and timely information to health care providers as part
of a comprehensive home care delivery model.
The technology developed is an automated behavioral monitoring system
(ABMS) that makes use of five motion detectors and a base station, connected
via the Internet, to a website that processes the sensor data and converts
them to behavioral information that is then displayed with graphics and
text for caregivers.
The system has been specifically designed to obtain a clear and accurate
knowledge of a person's performance of functional tasks in the home environment.
Within gerontology these functional tasks are termed Activities of Daily
Living (ADLS) and Instrumental Activities of Daily Living (IADLS). ADLS
are comprised of: bathing; dressing; using the toilet; eating; getting
in or out of bed; and walking while IADLS consist of: meal preparation;
managing money; grocery shopping; making a phone call; doing housework;
taking medication; and doing laundry. In other word, ADLs are focused on
one's personal care, e.g., being able to select clothing and dress and
undress without or with assistance, while IADLs are more focused on activities
that are associated with home management, e.g., being able to do laundry
or housework either alone or with some assistance. Underlying the assessment
of both of these sets of activities is the assumption that the inability
to successfully accomplish one or more of the individual activities jeopardizes
independence and wellbeing. Therefore, it is important to determine when
an individual is having difficulty completing one or more activities in
order that appropriate aid can be provided.
Although there are several widely used ADL/IADL assessment instruments
[Lawton, 1988; Kane, Kane, 1981;
Fillenbaum, 2001], each includes a combination of
observations and questions that ask the individual if they can successfully
complete a specific task with no help, some help or not at all. These assessments
are often supplemented by asking a caregiver the same questions about the
subject and, rarely, by asking the subject to complete a series of actual
tasks, e.g., walking around cones, opening jars. The information collected
by these assessment instruments is then used to help determine whether
the individual can function well enough to remain living independently
and with what level of help. In addition to serious questions concerning
the reliability and validity of these instruments [Wade,
Collin, 1988]--how wise is it to ask an individual who is having trouble
remembering things if they remember that they have forgotten to take their
medication--their greatest weakness stems from the fact that the ability
of elderly individuals to accomplish tasks declines over time. Consequently,
individuals have to be reassessed in order to determine changes in their
abilities, which raises costs as well as questions as to how often the
reassessment should take place.
Thus, during the 1990s it became obvious that some other method of assessing
a person's performance of functional tasks had to be found, a method that
increased accuracy, reliability and validity and allowed for frequent reassessments
so that more timely information was available. It was during this search
that attempts were made to employ various technologies in the assessment
of ADLS/IADLS, ranging from computerizing the paper and pencil instruments,
to placing video cameras in the residences of individuals. Even though
some of these attempts did provide better information, most efforts proved
either too expensive or too intrusive, or both. However, an approach that
used technologies derived from home monitoring and smart home applications
when combined with the Internet proved to be more successful in providing
greater accuracy, and more reliable and valid information, while at the
same time being cost effective and non-intrusive. This approach was automated
behavioral monitoring (ABMS).
2 ABMS Technology
The key development issues for any system that would automatically monitor
the behavior of frail older people in their own homes were its usability
and acceptability by both the individuals being monitored and by their
caregivers; it had to be easy to install and use, and it could not be threatening.
If a system required people to change their behavior, or it required major
modification of their residence or if they were fearful that the system
was "big brother", it would not be accepted and widely used.
Thus, eight principles comprising the overall design philosophy were developed
to meet these requirements: 1) the design should be based on the needs
of individuals, not on the existence of a technology; 2) it should use
existing technology, nothing new should be invented, but instead off-the-shelf
components used; 3) it should be invisible to the person being monitored;
4) its use should require no change in the normal behavior of the monitored
individual, it should be passive rather than active; 5) the installation
should require no retrofitting to the residence; 6) it should monitor objects
in the environment, not the individual; 7) it should be software driven
so that monitored activities could be easily added and removed as the needs
of the individual changed; and 8) it should be cost-effective, similar
to the cost of cable TV.
In order to achieve these design principles, technological features
were drawn from two main sources: smart-home and home automation
technologies; and telemedicine, especially physiological process
telemetry [Kutzik, Glascock, 2004]. With the
development of increasingly sophisticated ubiquitous computing systems
in the early part of the 21st century, it became feasible
for systems to respond to very subtle actions on the part of the
residents ranging from changes in gait to gestures to voice commands
[Himanen, 2004; Giroux, Guertin,
2004; Chan, Campo, Esteve, 2004]. These
technical improvements coupled with a growing interest in how to use
smart-home technology to help frail individuals remain safe and
independent has led to an emerging vision of the smart home which
plays a more active role by assuming functions traditionally provided
by human caregivers. Thus, the development of the ABMS drew
extensively from smart-home technologies that could be programmed to
remind the monitored individuals to turn off the stove, take their
medication, query them if they are alright, and, if need be, call for
help if there appears to be an emergency, such as a person falling
down and being unable to get up.
In addition, technologies associated with telemedicine and physiological
process telemetry proved to be extremely compatible with the design principles
underlying the ABMS. In particular, technologies developed by Japanese
researchers in the 1990s for collecting data on heart rate, blood pressure,
respiration, hydration and weight in the home environment proved to be
quite helpful [Togawa, 1992]. This work, along with
that of Alyufuku and Hiruta [Alyufuku and Hiruta, 1994]
on non-obtrusive means for collecting and analyzing physiological data
by embedding detectors in the home environment, adopted the same principles
as the ABMS of non-intrusiveness, passivity and no change in the behavior
of the monitored individual. Thus, an exercise bike becomes the means of
measuring and recording a variety of physiological performance data including
heart rate and calorie consumption, a bathtub becomes a means of measuring
at rest heart rate and a specially equipped toilet becomes a means of analyzing
urine and stool samples for possible pathological problems. This embedding
of monitoring devices in the home environment and the use of everyday behaviors
to provide the context of measurement anticipates several core features
of the technology that eventually became the ABMS.
Finally, it was apparent from research conducted on the views of a wide
range of individuals who worked with and cared for elderly individuals
that any monitoring system had to be able to monitor at least eight task
oriented behaviors:
1) medication adherence; 2) stove use; 3) eating; 4) overnight toileting;
5) wake up time; 6) general up-and-around; 7) bedtime and overnight sleep
disturbance; and 8) bathtub use [Glascock, Kutzik 2004,
In Press]. In addition, the research indicated that any effective monitoring
system had to be structured in such a way that monitored activities could
be easily added and/or subtracted depending on the needs of individual
clients, the context in which the system was installed, and changes in
the needs of the client over time. Monitoring bathtub overflow might be
essential in an assisted living facility, but not necessarily in a one
storey single-family dwelling. In other words, a cost effective system
had to be able to "switch on" and "switch off" activities
easily and quickly.
The resulting automated behavioral monitoring system consisted of two
main components: 1) a residential system consisting of motion detectors
and a base station; and 2) a website that provides for processing of the
sensor data converting them to behavioral information displayed with graphics
and text for caregivers. [Kutzik, Glascock, 1997;
2000a; 2000b]
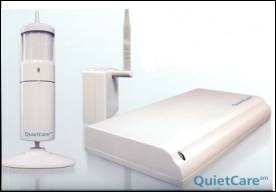
Figure 1: QuietCareSM ABMS Base Station and Motion
Detector
2.1 Residential system
The wireless motion detectors combine a passive infrared motion detector
with a 420 MHz wireless transmitter capable of reliably sending programmable
unit identification code for up to 120 feet to the base station. The base
station unit collects and transmits sensor data to the remote monitoring
site through the Internet. The base stations are comprised of a wireless
receiver, a microprocessor and flash memory for data storage, low level
processing and Internet upload control, plus a burst modem. The unit is
approximately 10 x 6 x 3 inches and is connected to the phone line through
a RJ-11 jack and plugged into a standard AC receptacle. The base station
firmware is designed to record and time stamp all incoming sensor signals,
store them in memory, format them to an XML file and upload periodically
to the web server via a burst modem at programmable time intervals.
To minimize the effects of power and/or phone line failure, the base
stations are designed to archive days or even weeks of data and to automatically
reboot and upload when power and phone lines are restored.
Although there is a standard protocol for detector placement, there
is often individual variation depending on the nature of the particular
residence into which the system is installed, as well as differences in
the behavior of the specific resident, e.g., two storey residence versus
one storey, resident sleeping in chair rather than bedroom. The most common
configuration of detector placement is: 1) in the bedroom facing the exit-doorway
in order to determine wake-up and bedroom activity; 2) inside the bathroom,
facing the exit door to determine entry into and activity in the bathroom;
3) outside the bathroom door to sense exiting of the bathroom; 4) either
inside the refrigerator or in the kitchen area facing the refrigerator
to determine meal activity; and 5) inside a box in which all medication
is kept. The decision to place a detector inside or outside of the refrigerator
is made based upon the nature of the kitchen, if general activity not associated
with meal behavior can be "picked-up" by the kitchen detector;
this detector is placed inside the refrigerator. The medication box/detector
array is a simple but robust way to determine if an individual has interacted
with her/his medication, since the detector registers each time the box
is opened. However, the data generated are not specific to any particular
medication, but only to the box being opened. Analysis of the data derived
from the opening of the box indicates that in approximately 90% of the
installations, this information is sufficient to know if an individual
is taking her/his medication. In the other 10%, a more sophisticated and
expensive "medication" system is required. No such medication
dispenser is currently part of the monitoring system, but several such
dispensers are available for purchase.
Several important conclusions about detector placement were reached
over the course of the development of the ABMS. First, detectors need to
be placed out of reach of people, especially individuals who use walls
to brace themselves when they move about their residences. Early in the
development several detectors were dislodged from outside the bathroom
by residents knocking them off as they walked down the hall. As mentioned
above, if a kitchen is more of a hallway than a separate room, placing
the detector on the wall records general activity rather than behavior
associated with meal preparation. Placing the detector inside the refrigerator
solved this data "confounding" problem. Finally, since the detectors
fit into a caddy that is attached to the surface, they can therefore be
rotated to face the appropriate direction. However, given the propensity
for individuals to rotate the detectors, it was determined that it was
best, once located, to permanently attach the detector to the caddy.
2.2 Web-based remote monitoring site
The web-based remote monitoring site consists of a PIN secured server
designed to receive behavioral data from the base units and translate them
into information. The server currently used is made up of: the web-based
front end for the caregiver and administrators; the SQL based relational
database management system; the automated scripts; and the Java based collector
software. The server operating system is a Red Hat Linux installation running
on Intel hardware. Once in the server, the raw data are translated into
information through the application of specific algorithms that take the
individual data points corresponding to sensor events and create composite
variables representing specific human activities.
Both status and trend analysis rules are used by the server. Status
rules analyze sensor events collected during the same day to infer if a
particular human event has occurred. For example, signals from the bathroom
motion detector are examined for their time stamp and compared with data
from the other motion detectors to determine if a person may have fallen.
Trend analysis rules, on the other hand, search for change in the state
of the present day's activity in relation to expected frequencies and timings
of activities based on a continuous baseline analysis. The information
that results from the application of these two rules is further distilled
to provide easily interpretable and timely advice to the caregiver.
Thus, the web site's PHP pages transform the information on each activity
into icons and clear narrative statements that relay the most important
trends as simply as possible. Current day information is automatically
compared to previously collected activity data in order to determine changes
in activity. Green indicators aqre displayed when the data show no significant
deviation in the frequency and/or timing of data for a given activity;
yellow status indicators are displayed when the frequency and timing of
a given activity is higher or lower than expected; and red status indicators
are displayed when a still higher threshold of deviation is recorded. Figure
2 is an example of an event summary page. Names used in this figure,
as well as in Figure 4 are pseudonyms.
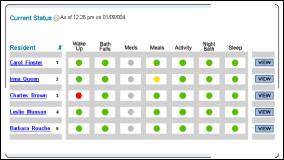
Figure 2: Event Summary Page
2.2.1 Determination of alerts
The monitoring system generates two different types of alerts: 1) emergency
for wake-up, falls and temperature; and 2) non-emergency for overnight
bathroom use, meal preparation and general activity. In the case of emergency
alerts, emails are sent at the time the system records the alert regardless
of the time of day. Only red alerts are generated for wake-up and falls,
whereas for temperature a yellow alert is generated for a predetermined
temperature and a red for an even higher or lower temperature. In the case
of non-emergency alerts, emails are sent by 10:00 am the day after the
status has been determined, e.g., the number of overnight bathroom visits
for the previous day were higher or lower than the predetermined threshold.
Only yellow alerts are generated for meal preparation, while both yellow
and red alerts are generated for overnight bathroom use and general activity.
On the advice of the medical advisory board, no alerts are sent for
medication adherence. However, the number of medication events is recorded
and available on the client's website, allowing for changes in medication
adherence to be observed and action taken.
2.2.2 Examples of how algorithms generate alerts
In order to show how the monitoring generates alerts, one example each
of an emergency and non-emergency alert will be discussed. A fall alert
is generated when the inside the bathroom sensor is triggered and the sensor
outside the bathroom is not triggered for at least 60 minutes. Once the
60 minute threshold has been passed an emergency alert email is sent to
the designated caregiver and the fall indicator on the client's website
turns red. The 60 minute threshold can be and has been adjusted to accommodate
the specific conditions of particular clients. In one case, the time period
was increased to 90 minutes because of the need for the individual to remain
in the bathroom for longer periods and reduced to 30 minutes for a second
client who was of greater risk of falls. A non-emergency alert for overnight
bathroom use is generated when the number of events during a 24 hour period
is higher or lower than a threshold based on a sigma value representing
a significant deviation from the expected value compared to a 30 day running
average of events. In other words, a yellow alert is generated for one
sigma value and a red for a second greater sigma value. The actual calculation
for the sigma values is proprietary, but the logic is straightforward.
2.2.3 What has been learned about thresholds
The first thing learned was that the thresholds for overnight bathroom
use and meal activity were too sensitive, thus generating, false positive
alerts that resulted in caregivers taking needless actions. After consultation
with the caregivers, this problem was easily corrected by adjusting the
sigma values used for overnight bathroom use and meal activity. The second
thing learned was more subtle, in some ways even more important and much
more of a challenge to correct than the adjustment of sigma values. Since
alert thresholds are based on deviation from an expected value equal to
the running average of the number of events for the last 30 days, the thresholds
are being continuously adjusted over time. Thus, changes in the number
of events for a specific behavior can be of long-term significance, but
because the changes are so gradual no alerts may be generated. Using overnight
bathroom activity once again, there can be a steady increase in the number
of events over a given 30 day period without producing either a single
yellow or red alert as the following series of real data points for a monitored
individual shows--2, 2, 3, 2, 3, 3, 3, 2, 4, 3, 3, 4, 4, 3, 5, 4, 4, 5,
4, 5, 5, 4, 5, 6, 6, 5, 6, 6, 5, 6. The system is obeying its own rules
for alert generation; however the change in the number of events, even
though too gradual to generate daily alerts, may be very significant for
the well-being of the monitored individual. Hence the conundrum: if the
thresholds are set to be sensitive to these gradual changes, too many false
positives are generated; however if they are not adjusted, no alerts are
generated and potentially serious health problems could be missed.
The solution was to develop a sub-routine that charted the average number
of actual events per day for each of the behaviors for a designated time
period--one week, two weeks, monthly. The sub-routine automatically counts
the number of events for each behavior, produces a daily average and creates
a multiple time period graph for each behavior.
Therefore, for the example above, the average for the previous thirty
days could be 2.2 while the average for the illustrated 30 days is 4.1.
The resulting graph would clearly illustrate the magnitude of the change
in the number of bathroom events between the two time periods and as a
result, allows for gradual and steady changes in behavior to be observed
even when no daily alerts were generated. A further discussion of these
trends charts is found in section 4.2 of this article
and a much more extensive discussion is found in Glascock and Kutzik, [Glascock
and Kutzik, In Press].
3 Field Test
To test the usability and acceptability of the ABMS, a field test was
undertaken in: 1) a high-rise, independent living senior building of one
and two bedroom apartments in New York City; and 2) independent households
in and around Philadelphia. A special PIN secured website was established
for each of the settings that allowed professional staff members and caregivers
access to the information on the volunteers on a 24/7 basis. The web pages
provided a daily summary for each of the volunteers for six activities:
waking time; bathroom falls; the taking of medication; meal preparation,
overall level of activity; and nighttime bathroom use. The volunteers were
interviewed prior to the installation of the monitoring system to obtain
information on demographic background, health status, self assessed functionality,
and formal and informal care. In addition, each volunteer was asked to
sketch a "typical" day's routine.
The characteristics of the volunteers from the two field sites differed
in several important ways. Although both populations were predominantly
African American (75%) and economically depressed--all but two individuals
living in subsidized housing--they differed considerably on average age,
79 for the volunteers at the high-rise, independent living senior building
in New York City, 68 for those living in and around Philadelphia, and gender,
80% male in Philadelphia, 80% female in New York. The most important difference
between individuals in the two groups was in level of impairment: the volunteers
in Philadelphia were much more impaired than those in New York and as a
result, 75% of the volunteers in Philadelphia experience impairments that
restrict their mobility, whereas only 20% of the volunteers in New York
experience such limitations. The field test ran in the New York City residences
from October, 2003 until April 2004 while it ran in the residences of the
Philadelphia area volunteers from March, 2004 until September, 2004.
3.1 Reliability and Validity
Results from the field test indicate that ABMS is extremely reliable,
produces valid information and is accepted by both the volunteers and their
caregivers. This is crucial because without a high level of reliability
and validity, the system will not meet the needs of the client or the expectations
of the caregiver. A large number of "false positives", i.e.,
indications that an individual did not wake-up, will result in needless
worry, disturbing phone calls or even the notification of emergency personnel.
Just as damaging are "false negatives", i.e., the lack of
the recording of an increase in overnight bathroom use, which could indicate
a change in health status that needs to be addressed. Consequently, one
of the main purposes of the field tests was to determine the overall reliability
of the system and validity of the information produced.
One of the main objectives of the field test was to assess the reliability
of the data generated by ABMS and overall these data were reliable at a
98% level. In order to conclude that ABMS itself operated reliably for
a single event the following had to occur: 1) the detector successfully
triggered; 2) the signal received by the base station; 3) the data recorded
and time stamped; 4) the data uploaded to the website; and 5) the information
depicted in the proper format. That is, that the color shown on the chart
[see Figure 2] was the result of the system operating
reliably. An example of an error is a red showing on the chart for "Bath
Fall" when no fall occurred. In this instance something went wrong
with the system: either the bathroom sensor recorded the participant's
entry into the bathroom, but not her exit; or the signal did not reach
the receiver; or the signal was not uploaded to the website. Over 12,500
such "events" were included in the analysis and the overall reliability
of the monitoring system was slightly over 98%. A second even more rigorous
test of reliability was employed in which every recorded "event"
for each day was examined to determine if there were inconsistencies, that
is, if an event was recorded, e.g., medication taken, when the detailed
activity chart indicated that there was no activity in the residence. If
the crosscheck showed such a contradiction, an error in the reliability
of ABMS occurred. This examination resulted in more than 47,000 data points
being cross checked and a reliability level of slightly less than 98%.
An attempt was made to determine the cause for the errors found in both
of the tests of reliability. The most frequent reasons in the first test,
accounting for 90% of the errors, was: 1) faulty sensor placement, e.g.,
placing a motion detector within the refrigerator to register each time
the refrigerator door was opened and closed did not work well resulting
in intermittent signals being received; and 2) human tampering, e.g., a
volunteer removing a motion detector from the wall because she did not
think that it was working. The most common cause of errors in the second
test of reliability was the appearance of activity, specifically meal preparation,
when no general activity was recorded.
These findings resulted in a series of small changes being made in the
way the system was installed and adjustments in several of the algorithms
that were used in the timing of general activity. First, more care was
taken during installation to discuss the need for residents not to move
the location of the motion detector or remove it from the wall, even if
it appeared to be malfunctioning. In addition, new detectors that actually
report when they are malfunctioning have been incorporated into the system.
Secondly, it was decided to use a stronger adhesive to attach the motion
detectors to the wall and, in many cases, to actually tape the detectors
to their brackets so that they could not be moved around. These two changes
eliminated most, if not all, of the reliability issues associated with
human tampering. As for the reliability problems associated with specific
behavior being reported without them being logged in the general activity
area, an adjustment to the algorithm that logged the various activities
corrected this aberration.
In contrast to the determination of reliability, it is more difficult
to present a precise level of validity of the information produced. This
is because of the nature of validity itself and the introduction of human
interpretation into the calculations.
In the context of our test, validity is regarded as the truthfulness
of the information produced by the monitoring system, that is, that the
system truly measures the selected activities. The system may be highly
reliable, but the information is only valid if it can be correctly interpreted;
that a red actually means that something is wrong.
To aid in our analysis, we have divided validity into two components:
machine validity and caregiver validity. Machine validity is a combination
of the system's reliability and the validity of the interpretive algorithms
that determine the patterns of behavior of the participants and continuously
look for change in the patterns. Thus, the colors displayed on the event
chart [Figure 2] are entirely the result of the monitoring
system's hardware and software functioning properly. It is reasonable to
argue that underlying this functioning is a set of assumptions about the
selected activities, e.g., the opening and closing of the refrigerator
is a valid "proxy" measure for meal preparation, but once these
assumptions have been programmed into the system, it is relatively easy
to determine the level of machine validity.
This level is 95%. Since, as stated above, the system's reliability
is a determinant of machine validity, it is, by logic, impossible for machine
validity to be greater than the systems reliability (98%); therefore, the
remaining errors must be the result of problems with the algorithms used
to produce the color indicators. All but a tiny number of these errors
are "threshold errors", e.g., the color indicator showing red
for meal preparation because of a decline in "events" from 22
the preceding day to 14. The algorithm that calculated the running average
was placing too much weight on the 22 events and not enough on the overall
pattern, thus resulting in the threshold for red to be crossed when it
should not have been. As a result, over 90% of the machine validity errors
were easily corrected by an adjustment in the appropriate algorithm.
Caregiver validity is not as easily calculated and yet, it is the reason
for the entire monitoring system to be developed. People, who are making
decisions based upon the "advice" that the system produces, must
have confidence that what they see is valid, or they will not take action.
If a caregiver is not completely confident that a red truly means that
an action must be taken, the system has little utility. The need to assess
caregiver validity led to a Pilot Study that focused on the way the ABMS
was actually employed in caregiving.
4 Pilot Study
A twelve month pilot study to evaluate the effectiveness of ABMS in
a comprehensive care management program began May 1, 2004. This study was
undertaken in conjunction with Keystone Home Health Services, Inc./Keystone
Hospice (Keystone) of Wyndmoor, Pennsylvania and Living Independently Group
Inc. (LIG) of New York City. Keystone provided access to their clients
and the participation of several of its visiting nurses, while LIG allowed
the use of QuietCaresm, their commercialized product based on
the ABMS. The goal of the pilot study was to have systems operating in
the homes of twenty individuals with chronic health care problems for twelve
consecutive months. However, this has proven to be difficult because of
a higher attrition rate than originally anticipated. Although QuietCaresm
has been installed in the residences of a total of twenty-six Keystone
clients, currently the system is operating in only seventeen.
Three of the original clients have died, another three have had to enter
institutions because of greater care needs and Keystone has discontinued
services to another three. In addition, three of the clients still in the
pilot study have spent periods of two to five weeks in the hospital, while
another two have been unable to pay their phone bills for at least one
month. The result of this is that the system has not been running consecutively
in any of these five residences.
This higher than expected attrition rate is the result of the seriousness
of the health problems of the clients and their economic status. Each of
the clients, regardless of age, have a multitude of health problems, including
among others, congestive heart failure, emphysema, HIV, Parkinson's, Alzheimer's,
diabetes, cancer and LUPUS. In addition, the level of poverty experienced
by many of the clients in the study has not only been a contributing factor
in their overall health, but also in their ability to maintain an operating
phone, that is essential for the operation of the monitoring system. Thus,
new clients have been added as others have been removed and the duration
of the pilot study has been extended to eighteen months. In this way, it
is hoped that a total of twenty clients will have the system in their homes
for twelve months; it's just that they will not be the original twenty
clients.
The characteristics of the twenty-six clients who have been, for any
length of time, participants in the pilot study are as follows: seventeen
are male and nine are female; seventeen are African-American, seven Caucasian,
one Hispanic and one Asian-American; the average age is 57, with six clients
over the age of 65; all but two are economically disadvantaged; and all
but one lives in the city of Philadelphia.
4.1 The Caregiving Pyramid
The challenge associated with the introduction of any new caregiving
practice, let alone one dependent on the use of an innovative technology,
is how to engage practitioners and clients to use it. Even if practitioners
are willing to try to use it, and clients willing to have it in their homes,
an equally daunting challenge is to incorporate the new technology into
existing caregiving practice models. Professional caregivers often fear
that they will be replaced by a machine and/or dread appearing dim-witted
because they are unfamiliar with the technology. Clients, on the other
hand, are often fearful of new people and machines entering their homes,
especially machines that "report" on their behavior. Thus, it
is imperative that the caregivers, as well as the clients who have QuietCaresm
in their homes, understand the role played by behavioral monitoring in
a comprehensive care program. This role was outlined in the introduction
of this paper: to obtain clearer and more accurate knowledge of a person's
performance of functional tasks in their home environment. This is all
that ABMS does; it just does it more reliably, with a greater level of
validity and in a timelier manner than other such methods. In order to
explain how this is accomplished within the pilot study, it is necessary
to understand how data and information are used in caregiving.
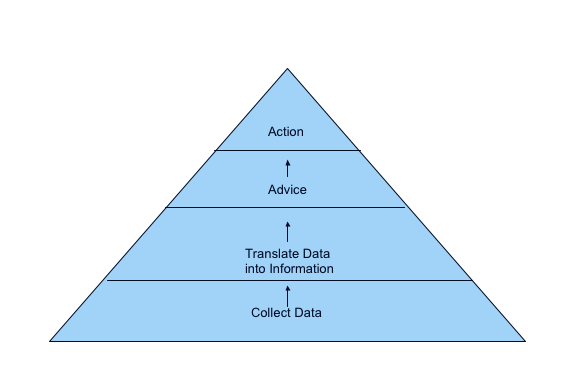
Figure 3: Caregiving Pyramid
The above figure depicts a general caregiving model that begins, at
level one, with the collection of data on a client. In this context, these
data are about functional tasks, e.g., overnight toileting, medication
adherence. As discussed in the Introduction, in existing caregiving models,
these data are most often collected by various forms of retrospective interviewing,
supplemented by pill counting or questioning a caregiver about the client's
behavior. The reliability of these data is always a concern because of
timeliness and the accuracy of responses. If the data are unreliable, the
information that results, level two of the pyramid, will not be valid.
Validity entails the human interpretation of the data and, in a caregiving
context, is usually the result of some protocol combined with experience.
In other words, the data, whether reliable or not, are subject to interpretation
that translates the data into information. An example of invalid information
is an alarm generated by a personal emergency response system indicating
that there is a fall when no fall has occurred. This is a false positive
and is both expensive and dangerous, given that frequent such false positives
could result in the assumption that there is no emergency and thus lack
of response.
However, all information, in a caregiving context, is ultimately distilled
into some form of advice-level three on the pyramid. This advice may be
quite specific, as illustrated in the fall example above, a client has
fallen, or more general, medication needs to be reviewed with the client
because there are too many pills left at the end of the month. Whether
general or specific the advice leads directly to action, level four, by
the caregiver-phone 911, speak to client about medication adherence. The
action is taken because the caregiver is confident in the advice, the advice
is regarded as accurate because the information on which it is based is
valid, and the information is valid because the data are reliable. Thus,
we have come full circle. If the data are not reliable, the base of the
pyramid, the action taken by the caregiver may not be appropriate or the
timing may be inappropriate or the caregiver taking the action may not
be the most appropriate person for the required action.
ABMS was designed to collect more reliable data and to more effectively
and efficiently translate the data into valid information than other data
gathering techniques. The field test, reported upon above, has demonstrated
that the system collects extremely reliable functional data (98% level
of reliability) that compares favorably with data collected by any other
caregiving model.
In addition, the data are much timelier, being collected and analyzed
continuously compared to the infrequent data gathering devices employed
in other caregiving models. Moreover, the resulting information has an
extremely high validity (95%) and an undeniable advantage over the information
collected by any other caregiving model. The information generated is continuously
interpreted, both on a daily basis and through trend analysis, to pinpoint
changes in behavior that could indicate a problem before it can become
a crisis. The key, of course, is how this information is distilled into
advice and then action taken by the caregiver.
4.2 Alerts and Trends
QuietCaresm provides two types of information for caregivers:
1) alerts; and 2) trends. The alerts, as discussed previously, are either
red or yellow and "alert" the caregiver to a significant change
in one or more of the monitored activities, e.g., decrease in meal activity,
increase in overnight bathroom use, or that a fall has occurred. In the
pilot study, the visiting nurses received emails indicating an alert and
through this email they had access to the client's specific website so
that they could check other activities. Trends, on the other hand, are
the result of the analysis of data over a much longer period of time. In
the pilot study, a monthly average was generated for each of the activities
monitored: a client could average 3.5 overnight bathroom visits, 22.25
meal activites, and 2.3 medication events, for example, per day, for the
month of July. These monthly figures could then be "plotted"
for several months so that trends could be discerned.
Figure 4 is an example of an alert received by a
visiting nurse during the pilot study. The red disk indicates that a fall
has occurred while the two yellows specify that there has been a significant
decline in the number of meal events and overall activity during the previous
24 hours. The caregiver can and did receive more detailed information about
these activities, time of the fall, actual number of meal events and level
of activity, by clicking on the colored disk.

Figure 4: Alert Line
Thus, alerts notify a caregiver as to significant changes in the activity
level of the client during the previous 24 hours and thus, allow for action
to be taken to address the indicated problem almost immediately. Trends,
since they are based on the analysis of data from a much longer period
of time, allow for more subtle changes in behavior to be observed. These
are the type of gradual and slight changes that are difficult, if not impossible,
for the individual themselves to notice or even for a caregiver to discern.
Retrospective interviewing as to changes in the level of activities over
the last month, six months, year, even by a well meaning and dedicated
caregiver, is fraught with difficulty and the results with uncertainty.
QuietCaresm, on the other hand, provides objective data that
is translated into timely and accurate information and then charted to
provide clear and easily deciphered trend summaries.
Figure 5 shows a steady decline in meal activity
over a six month period for an elderly African American woman. Even though
only a few meal alerts (7 in over 180 days) were generated during this
six month period because the day-to-day changes were too slight to register
as significant and thus to generate an alert, Figure 5
clearly shows a steady decline in meal activity from an average of 23.2
in May to 8.3 in September. As it turns out, the decline in meal activity
confirmed the overall impression by the visiting nurse and client's physician
of decline and the client was moved to an assisted living facility at the
end of September.
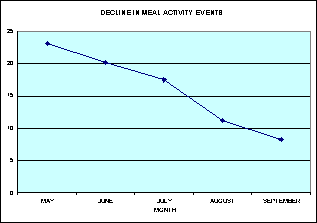
Figure 5: Trend Chart
4.3 Case Studies
The following seven case studies are drawn from the over one hundred
times that the visiting nurses have used an email, an alert, the website
and/or monthly trend chart to alter the care provided for twenty-two of
the twenty-six clients (85%) with QuietCaresm in their residences.
All but two of these twenty-two clients have had their care altered more
than once, with three of these clients having their care altered over ten
times each. The phrase "alter the care" actually entails one
of nine actions that can be triggered by information produced by the system:
1) increase in the frequency of home visits by nurse; 2) reduction in the
frequency of home visits by nurse; 3) unscheduled phone call; 4) unscheduled
home visit; 5) adjustment in care plan, e.g., medication counseling; 6)
bringing to the attention of other care providers that needs of client
had changed; 7) immediate response, e.g., absence indicated, fall alert;
8) increased level of care outside of residence, e.g., hospitalization,
other institutionalization; and 9) non-medical/clinical action, e.g., home
health aide not at residence, air conditioner repair.
Thus, each alteration of care is actually an episode comprising three
distinct parts: the trigger-an email, alert, monthly trend chart;
the action-an unscheduled phone call, an immediate response; and
an outcome-medication counseling, adjustment of nurse's schedule,
hospitalization.
Some of these episodes have only a single action and a single outcome,
i.e., an unscheduled phone call that results in the nurse being reassured
that the client is stable and that no other action is required, while others
involve multiple actions and multiple outcomes, i.e., an unscheduled phone
call, resulting in an unscheduled visit, resulting in notifying other care
providers that the client's needs have changed, leading to hospitalization.
4.3.1 Case Study 1
The first case study describes a single episode in which the visiting
nurse received a red alert email indicating a dramatic increase in overnight
bathroom use (three times normal) for a 48 year old African-American male
suffering from renal failure. The nurse, following the Keystone/QuietCaresm
protocol, made an unscheduled phone call to the client to inquire as to
the reasons for this spike in overnight bathroom use. The client told the
nurse that he had been up all night treating a bad case of hives and that
he had been in and out of the bathroom several times. Since the client
also suffered from HIV and thus, the hives could lead to a serious infection,
the visiting nurse phoned the client's physician to discuss possible treatment
options. Once a decision had been made as to the best treatment, the nurse
once again phoned the client with the information and instructions as to
how to obtain the medication and how to use it. The following day the visiting
nurse called-up the client's webpage and was reassured that the client
had returned to his normal overnight bathroom use pattern indicating that
the treatment for the hives had been effective.
4.3.2 Case Study 2
The second case study also describes a single episode, but this time
the visiting nurse received a red alert email indicating that there had
been a dramatic decline in general activity over the last 24 hours of a
67 year old African-American male suffering from congestive heart disease.
The nurse quickly checked the client's website and determined that there
had been no activity recorded since early the previous evening. Once again,
following the Keystone/QuietCaresm protocol, the nurse made
an unscheduled phone call to the client. When the client did not answer
the phone, the nurse made an unscheduled visit to the residence of the
client, where he discovered that the client was unconscious. The nurse
notified the appropriate emergency service and the client was immediately
taken to the hospital for treatment of the heart attack he had suffered.
4.3.3 Case Study 3
The third case study, although describing a single episode, is carried
out over several days and shows how behavioral monitoring, in addition
to indicating the need to immediately respond to an emergency situation,
can be used to track changes over a period of time. In this case the, visiting
nurse received yellow alert emails indicating that for two straight days
a 43 year old Caucasian male suffering from metastatic cancer of the bladder
and bowel had only one-half of his normal meal events-from an average of
over eight per day to only four events. In addition, on the second day,
for the first time in two weeks, the client had not taken his medication.
The nurse, following protocol, made an unscheduled phone call to the client
to determine his status, the client stated that he felt bad, but didn't
think that it was necessary for the nurse to make an unscheduled visit.
However, the next morning the nurse received two yellow alerts for this
client: one indicating that meal activity had declined to only two events;
and the second indicating that the client's activity level had declined
to only 34% of normal. These alerts, combined with the fact that for the
second straight day the client had not taken his medication, resulted in
an unscheduled phone call to the client followed by an unscheduled visit
at which time the nurse determined that the client, who had been vomiting
and experiencing diarrhoea for the previous three days, needed to be hospitalized.
4.3.4 Case Study 4
The fourth case study consists of two closely related episodes concerning
a 73 year old Caucasian female suffering from Parkinson's disease and hypotension.
The visiting nurse received an email red alert indicating a client had
a fall and yellow alerts for meal activity that had declined from eleven
the previous day to three on the day in question and general activity that
was only 23% of normal on the given day. The nurse made an unscheduled
phone call to the client and when she did not answer the phone the nurse
immediately went to her house. She had had a fall, had made it to her bed,
but was disoriented and in pain. She was admitted to the hospital. Tellingly,
the client was wearing a pendant that was part of a personal emergency
response system when she had her fall. When the visiting nurse asked her
why she hadn't pushed the button on the pendant, she replied "I didn't
want to bother anybody. I was able to get back to bed, so I thought I was
ok".
Less than three weeks after the client had been discharged from the
hospital, the visiting nurse received a red alert email indicating, once
more, that the elderly client had had another fall while making a trip
to the commode at approximately 10:30 pm. Again, the nurse made an unscheduled
phone call, followed by an unscheduled visit to the client. Although she
was well enough to remain in her own residence, given her frail condition,
the nurse brought her condition to the attention of her physician and began
to check the client's web page daily, even if no email alerts were received.
When the client had another fall three weeks later at almost the same time
of night, the nurse once again made an unscheduled phone call followed
by an unscheduled visit with the result being that this time she was admitted
to the hospital. After reviewing her case with her physician, it was determined
that the client was taking her pain medication too close to bedtime with
the result being that when she got out of bed to use the commode, she was
woozy and subject to falling. Given that the client made a trip to the
commode almost every night between 10:00 pm and 11:00 pm, the physician
recommended that she take the pain medication earlier in the evening which
would reduce the possibility of her being woozy and falling. Since this
suggestion was made, the client has not fallen.
4.3.5 Case Study 5
Less than two weeks after a 54 year old Hispanic male client returned
from the hospital, the visiting nurse received an email red alert indicating
the client had two falls during the night. The nurse made an unscheduled
phone call to the client who appeared confused on the phone. The nurse
made an unscheduled visit during which the client denied having a fall
and reported that he had felt bad and was constipated so he had spent "long"
periods in the bathroom which, because these events were over an hour in
duration, QuietCaresm interpreted as "falls".
Even though the nurse recommended an over-the-counter remedy for the
client's constipation, over the next eight days, a total of nine red alert
fall emails were received and each day the nurse phoned the client who
stated that he had not fallen. During this same period, the nurse received
four yellow and one red email alerts indicating increased overnight bathroom
activity. The nurse phoned the client on each of these days and made unscheduled
visits on three of the days. The client's overall decline, as reflected
in the fall alerts, increased overnight bathroom use and confused state-of-mind
resulted in the client being moved into a hospice facility.
4.3.6 Case Study 6
In the sixth case study, the visiting nurse received a red alert email
that there had been no wake-up for a 49 year old Caucasian female client
suffering from LUPUS, severe depression and moderate dementia. Checking
the client's web-site, the nurse determined that there had not been any
general activity overnight which for this client was extremely unusual
since she had a consistent pattern of disturbed sleep that was indicated
by frequent overnight activity. When an unscheduled phone call to the client's
residence was not answered, relatives were notified. Fearing that the client
had had a fall or was in some other way incapacitated, a relative went
to the residence and found that the client was not there. It turns out
that the client had made an unplanned visit to her daughter who lived out
of town and thus, the client was not at risk. However, the nurse, client
and relative all expressed relief that the nurse had been alerted to the
client's absence and that the Keystone/QuietCaresm protocol
had been followed and someone had been sent to check on her well-being.
4.3.7 Case Study 7
Over a seven day period, the nurse received four email alerts concerning
medication adherence for a 51 year old African American male with heart
disease, hypertension and renal failure. A review of the client's web page
showed a dramatic fluctuation in the number of times each day the client
took his medication, some days the client did not take his medication at
all and on other days it appeared as if he was taking it six or seven times
a day. Based on this information, the nurse increased his home visits from
once a week to twice a week and began to personally fill the client's medication
dispenser. These changes, when accompanied by discussions on the importance
of following the prescribed medication regime, resulted in the client's
medication adherence becoming consistent and, over time, to an actual reduction
in the frequency of home visits by the nurse to once every two weeks.
4.3.8 Case Study Summary
In each of the above case studies, the system provided information on
the status of the individual client that was then used by the care provider
to take an appropriate action. These actions ranged from unscheduled phone
calls to check the client's status, to adjustments in the client's care
plan, to immediate responses resulting in hospitalization. However, in
each case, the care provider responded based on their confidence that the
emails they received, confirmed by the information on the client's web
pages, indicated that a change in activity had occurred that put the client
at risk.
That is, that the data collected, level one of the pyramid, was reliable,
that, in turn, this information received by email or viewed on the web
page was valid, level two, advice found in the protocol was appropriate,
level three, and the action necessary, level four. The result of the action,
in each and every one of the case studies was that the client received
more appropriate and timely care and thus, had greater well-being.
5 Conclusion
The case studies illustrate the means by which smart-home technology,
health telematics and the Internet can be used for the assessment of the
functional abilities of individuals in their own homes within the community.
Instead of the frail individual having to visit a health care facility
or a caregiver having to make repeated visits to the residence, QuietCaresm
continuously collected and interpreted the clients' activities of daily
living. This information is then used by the caregiver to alter care and
respond to emergency conditions. The case studies make clear that the clients
received and continue to receive better care and, as a result, are able
to retain more independence while at the same time, have a greater sense
of well-being. The following three statements exemplify the feelings that
many of the clients have about the ABMS and the way that it helps provide
care to them:
Just knowing that someone knows if I am in trouble makes me feel safer.
I have several friends who really need the system too. (elderly female
client)
This is a great idea. It's really like a friend who checks on you each
day. When I was really sick once, I didn't do what I normally do, but it
took several days before a friend of mine noticed that I wasn't at group.
Then she checked on me. No one in the apartments even noticed that I wasn't
around. Now, I know that someone is checking on me daily. This is great.
(female client)
It's like someone out there looking after me, making sure that everything
is OK. Since I have lost most of my equilibrium because of my problem with
my hearing, I am really worried about falling. This way, Tim can check
on me to see that I have fallen or am not able to move about. (male client
with Meniere's disease)
Additionally, the visiting nurses who are using the system to help them
provide care to their clients also have favorable opinions:
Since I make a phone call to at least one of my clients using the system
daily, I feel much better about the level of care I am delivering because
I have a specific issue that I can address, rather than just 'how are you
doing'.
I believe that my relationships with my clients who have the system
have become closer. They feel more connected to me and to the treatment
they receive from me.
Thus, QuietCaresm allows for more appropriate care to be
provided by more appropriate caregivers in a timelier manner than other
care provision models, because it focuses on specific task oriented behaviors.
However, behavioral monitoring alone provides only part of the total care
package that frail individuals require if they are to remain in their residences.
It is apparent that many of these individuals also require services that
can best be provided by telemedicine and additional environmental and security
functions most often found today in smart-home applications. Consequently
it appears logical that behavioral monitoring, telemedicine and certain
environmental and security functions be integrated or bundled into a much
more comprehensive system.
The first steps in this bundling have taken place as part of the pilot
study discussed above. The recording of ambient temperature is now part
of the QuietCare system and red and yellow alerts are sent to the visiting
nurse if the temperature in a client's residence is too high or too low.
In at least two instances, the nurse has responded when the temperature
was dangerously high and in both cases, repairs were made to air conditioners
on behalf of the client. Plans have been made for additional environment
and security features to be added to the QuietCaresm system
including sensors that indicate when the front door is opened, smoke detectors
and a stove monitoring device. Commercially available telemedicine features
are being also bundled with the system. These include: a blood pressure
cuff; a digital scale for recording and monitoring a client's weight; a
blood sugar monitor for diabetics; and sophisticated medication dispensers.
Finally, a personal emergency response system (PERS) is being included
to provide an additional level of security.
The plan is to integrate these various components into a single bundled
system that could be used as easily and effectively as the current iteration
of QuietCaresm. Once this new system is functional its testing will necessitate
a larger and more comprehensive demonstration project than the currently
on-going pilot study. However, the same measures will be employed to evaluate
the bundled system that are being used in the pilot study. In other words,
is the system able to provide better care, to a larger number of individuals
living in their own residences in a cost-effective way, than existing care
provision models? If the bundled system can achieve these objectives, then
the full potential of health telematics, and in particular pervasive computing
technology, will have been met.
Acknowledgements
We want to thank John Lakian and George Boyajian, Ph.D. of Living Independently
Group, Inc, New York City, for the material presented in Photograph 1 and
Figure 1. In addition, we want to thank our partners
in the field test and pilot study at Keystone Home Health Services, Inc./Keystone
Hospice in Philadelphia and Phelps Home Residence at Goddard-Riverside
Settlement in New York City. In particular, we thank Gail Inderwies, Louise
Taft, Michael John and Tom Salber from Keystone and Doris Colon at Goddard
for their participation in the project and their many contributions to
our understanding of comprehensive care management.
References
[Alyfuku, Hiruta, 1994] K. Alyfuku, Y. Hiruta: "Networked
Health Care and Monitoring System"; United States Patent # 5,410,471,
United States Patent Office, Washington, DC, USA, 1994.
[Chan, Campo, Esteve, 2004] M. Chan, E. Campo,
D. Esteve: "Monitoring Elderly People Using a Multisensor System";
Toward a Human-Friendly Assistive Environment. D. Zhang, M. Mokhtari, editors,
Assistive Technology Research Series 14, IOS Press, Amsterdam, The Netherlands
2004, pp. 162-170.
[Fillenbaum, 2001] G. Fillenbaum: "Multidimensional
Functional Assessment: Overview"; The Encyclopedia of Elder Care,
M. D. Mezey, editor-in-chief, Springer, New York, USA 2001, pp. 438-440.
[Giroux, Guertin, 2004] S. Giroux, S. Guertin: "A
Pervasive Reminder System for Smart Homes"; Toward a Human-Friendly
Assistive Environment. D. Zhang, M. Mokhtari, editors, Assistive Technology
Research Series 14, IOS Press, Amsterdam, The Netherlands 2004, pp. 98-106.
[Glascock, Kutzik, 2004] A. P. Glascock, D. Kutzik:
"Moving Telematics from the Laboratory to a Truly Enabling Technology
within the Community"; Toward a Human-Friendly Assistive Environment.
D. Zhang, M. Mokhtari, editors, Assistive Technology Research Series 14,
IOS Press, Amsterdam, The Netherlands 2004, pp. 145-154.
[Glascock, Kutzik, In Press] A.P. Glascock, D.
Kutzik: "Automated Behavioral Monitoring." In Smart Homes and
User Values, edited by U. Keijer and G. Sandstrom. Eastern Mediterranean
University, Faculty of Architecture, Gazimagusa, Mersin, Turkey, In Press.
[Himanen, 2004] M. Himanen: "Potential for
Smart Home Technology"; Toward a Human-Friendly Assistive Environment.
D. Zhang, M. Mokhtari, editors, Assistive Technology Research Series 14,
IOS Press, Amsterdam, The Netherlands 2004, pp. 67-78.
[Kane, Kane, 1981] R. A. Kane, R. L Kane: "Assessing
the Elderly: A Practical Guide to Measurement"; Lexington Books, Lexington,
MA, USA 1981, pp. 25-67.
[Kutzik, Glascock, 1997] D. Kutzik, A. P. Glascock:
"System for Generating Periodic Reports, Generating Trend Analysis,
and Intervention in Accordance with Trend Analysis from a Detection Subsystem
for Monitoring Daily Living Activity"; United States Patent #5,692,215.
United States Patent Office, Washington, DC, USA, 1997.
[Kutzik, Glascock, 2000a] D. Kutzik, A. P. Glascock:
"User Monitoring System"; Canadian Patent #2,208,594. Canadian
Patent and Intellectual Property Office, Ottawa-Hull, Canada, 2000.
[Kutzik, Glascock, 2000b] D. Kutzik, A. P. Glascock:
"System for Generating Periodic Reports, Generating Trend Analysis,
and Intervention in Accordance with Trend Analysis from a Detection Subsystem
for Monitoring Daily Living Activity-2"; United States Patent #6,108,685.
United States Patent Office, Washington, DC, USA, 2000.
[Kutzik, Glascock, 2004] D. Kutzik,
A. P. Glascock: "Monitoring Houshold Behavior to Enhance Safety
and Well-Being." In Gerotechnology: Research and Practice in
Technology and Aging, David Burdick and Sunkyo Kwon, editors,
Springer Publishing Company, New York, NY, pp. 132-144.
[Lawton, 1988] M. P. Lawton: "Scales to Measure
Competence in Everyday Activities"; Psychopharmacological Bulletin,
24 1988, pp. 609-614.
[Togawa, 1992] T. Togawa: "Physiological Monitoring
Techniques for Home Health Care"; Biomedical Sciences and Instrumentation,
28 1992, pp. 105-110.
[Wade, Collin, 1988] D. T. Wade, C. Collin: "The
Barthel ADL Index: a Standard Measure of Physical Disability?"; International
Disability Studies, 10 1988, pp. 64-67.
|